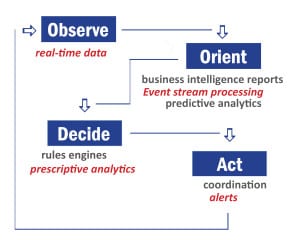
Before rushing to buy data analytics software, companies should consider what kinds of business decisions they want to make. That will lead to the data.
With growing amounts of data from every source imaginable—IoT sensors, e-commerce, financial transactions, and healthcare monitoring—many businesses are looking to make a quick buck in real-time data applications.
Not so fast. Experts advise that companies start with business process management — which considers operations and business outcomes, along with decision management.
In a recent white paper, James Taylor, CEO of Decision Management Solutions, noted that analytic technology has become increasingly easy to use, powerful, and comes with stunning visuals. “This makes it easy for teams to start with a visualization or data mining tool to solve a problem without defining a business problem first,” he stated. “The business results of such an approach generally disappoint.”
Of course, for businesses that can do it well, there are any number of successful use cases for analytics tools. Amazon and Netflix, for example, make a good portion of their sales profits from recommendation engines that use customer-relationship management systems and real-time data from clickstreams, all of which are folded into predictive algorithms to present customers with the “next-best” product or movie.
There are also a slew of industries that have met with success in using data for applications such as predictive maintenance, energy production, and financial compliance.
Related: Case studies from the energy, financial services, and healthcare sectors.
Using a Decision Management Model
Taylor advises that by building a decision requirements model and defining decision-making first, analytic teams also discover what data might be useful—which is to say, the data that matters most to the business decision that needs to be made.
Before embarking on an analytics project, Taylor recommends use of the new industry Decision Model and Notation (DMN) standard which was approved in 2015 by the Object Management Group. Decision Management Solutions is a submitter of the DMN standard along with Escape Velocity, FICO, IBM and Oracle, and co-authors KU Leuven, Knowledge Partners International, Model Systems and TIBCO.
According to the standard, its purpose is to provide constructs needed to model decisions so organizational decision-making can be readily depicted in diagrams, accurately defined by business analysts, and automated (optional). The standard addresses decision-making from two different perspectives: business process management models, and decision logic, for example packet-reception ratio (PRR) and predictive model markup language (PMML).
For advanced analytics such as data mining or predictive analytics, Taylor advises using the Cross-Industry Standard Process for Data Mining (CRISP-DM), the most widely used approach, which focuses on business understanding first. Another approach is SEMMA, created by SAS. Differences between the two methodologies can be found here.
Making Decisions: 7 Kinds of Operational Intelligence
Developed by USAF Colonel John Boyd as a way to model dogfights, the OODA Loop stands for observe, orient, decide, and act. A Gartner webinar by W. Roy Schulte shows how the loop correlates with operational intelligence, real-time analytics, and business process management:
In the orient phase, there are three tools: business intelligence and business activity monitoring (BAM) reports, along with event stream processing and predictive analytics. A business intelligence system might include something like Tableau or Tibco software. Data discovery and root-cause analysis are often performed at this point.
Event stream processing could deal with a variety of different data types, depending on the industry. For instance, the flow of financial transactions is constant and a stream could take into account A.T.M activity; retail, travel, and hospitality sectors may get streams from social media; e-commerce might take in information from clickstreams; and of course, there is the fast-growing segment of streams from machine data, such as IoT sensors. Schulte noted that there’s a difference between event stream processing and complex event processing, with CEP drawing higher level conclusions with multiple sources of data, such as with fraud detection. (Another example is collision avoidance–see our white paper on “Approaches to Complex Event Recognition”).
Predictive analytics make use of statistics, regression models, and other kinds of probabilistic reasoning to forecast what might occur—one example is sentiment analysis, such as how customers feel about a brand, forecasting supply chain issues, or scoring credit.
In the next step, decide, a business rules engine or software system uses decision trees, tables or other representational language. In this phase, prescriptive analytics, which can use machine learning, may attempt to answer what should be done, with one example being the presentation of a “next-best” offer in the e-commerce sector, or predictive maintenance.
For the final phase, it’s all about workflow coordination and getting the right message to the right person at the right time (if indeed a person is making the decision).
“If you want to improve how business decisions are made, you look for the weak link in the chain,” Schulte advised.
Want more? Check out our most-read content:
Research from Gartner: Real-Time Analytics with the Internet of Things
The Value of Bringing Analytics to the Edge
Frontiers in Artificial Intelligence for the IoT: White Paper
Data Visualization: How a Futures Exchange Sees Clearly
John Bates, Plat.One: Enterprise IoT Doesn’t Have to Be Hard
Why Edge Computing Is Crucial for the IoT
Why Gateways and Controllers Are Critical for IoT Architecture
Liked this article? Share it with your colleagues!