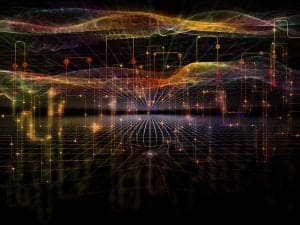
Data consumption is a critical part of the data lifecycle, and with the introduction of new tools, it increasingly benefits from automation.
In May of this year, we published an article entitled Improve Data Lifecycle Efficiency with Automation, where we opened the discussion around how the identification, collection, integration, and utilization of information in its various forms has accelerated in recent years. We covered the broad strokes of the data lifecycle and then went deeper into each aspect of the lifecycle in subsequent articles (Automation of the Data Lifecycle: Focus on Data Creation and Automation of the Data Lifecycle: Focus on Data Storage that also covered aspects of Data Quality.) This piece will focus on an area that has seen some of the most dramatic changes and one that is of critical importance to the primary business users of data – Data Consumption.
The data consumption layer is the area of the data lifecycle that sits between the sources of one’s data and the users of that data. This is an area that has seen some significant advances in recent years with the release of commercially available tools that help today’s data-centric leaders run their organizations in a more proactive way. Not too long ago, data professionals leveraged reporting tools and early-stage business intelligence solutions that offered visibility into what had and (in some cases) what was happening within the organization. This offered tremendous value and allowed us to make sense of the growing volumes of data (largely) within the confines of the organizational firewalls (e.g., Microsoft Excel typically used to organize data and perform financial analysis.) As technology advanced and the thirst for more proactive analysis grew, new tools and solutions allowed the data consumer to be more proactive and gave birth to predictive and prescriptive solutions – turning data into information and information into actionable intelligence.
Below we will focus primarily on some of the more recent advances in data consumption and how automation of this aspect of the data lifecycle provides significant value to the business. Traditional analytics and business intelligence, while still of value, are being supplemented and even replaced by tools and solutions that augment (and even replace) the human in the equation. Augmented analytics and self-service solutions driven by AI-enabled bots have become more widely leveraged and in demand given the distributed workforce and our hunger for information. First, we will look back and explore some of the more widely used legacy tools and solutions from the early days of reporting and business intelligence and then showcase some of the fantastic and game-changing offerings available today.
One cannot really talk about reporting and analytics without mentioning Microsoft Excel. Excel is the most widely used data analytics software in the world and is the tool of choice for many business professionals. It is the most common tool used for manipulating spreadsheets and building analyses and has been for decades. Excel is installed on most business and personal computers, is easy to learn, easy to use, and provides fantastic visualization capabilities for reporting and analytics. While Excel offers powerful reporting and (descriptive) analytics capabilities, an organization looking for deeper insights that span multiple sources and types of data might have looked to solutions offered from some of the large enterprise solution giants like SAP, Oracle, or IBM. For example, BusinessObjects (BOBJ), which was acquired by SAP in 2007, offered/offers clients an enterprise-ready solution for reporting and analytical business intelligence (BI) that aided users to find data, generate canned or custom reports, and conduct deep analytics across multiple data sources. These (now) legacy solutions have matured over time, and many have morphed into next-generation offerings leveraging Artificial Intelligence (AI) to automate the acquisition and analysis of data aiding the data professional seeking more real-time, forward-looking insights.
Today’s data-centric leaders are no longer satisfied having to rely on others to feed their hunger for insights and require more immediate gratification from their data. Traditional/legacy analytics offerings have, therefore, seen significant disruption recently by platforms that leverage AI to augment the human interaction and automate much of the data discovery, acquisition, and analysis – many leveraging bots and virtual assistants that are conversational in nature (such as Tableau and Microsoft Power BI.) This provides the (business) data professional with more of a self-service type offering and reduces some of the reliance on IT. In Q4 2019, Microsoft released Automated ML in Power BI. According to documentation from Microsoft, with Automated ML, business analysts can build machine learning models to solve business problems that once required data scientist skill sets. Power BI automates most of the data science workflow to create the ML models, all while providing full visibility into the process. Power BI is a collection of software services, apps, and connectors that work together to turn your unrelated sources of data into coherent, visually immersive, and interactive insights. Your data may be an Excel spreadsheet or a collection of cloud-based and on-premises hybrid data warehouses. Power BI lets you easily connect to your data sources, visualize, and discover what’s important, and share that with anyone or everyone you want. As organizational leaders thirst for deeper, more real-time, forward-looking insights has increased, so has the demand for these types of solution offerings.
Regardless of the type of technology you are using or the stack that you currently have in place, automation is playing a larger role in the ability to get to the information one needs to make an informed business decision. Take reporting as an example. At one point, it was necessary to run an extract on the data to get what one wanted in a usable format. One, then, needed to understand the structure of the data to ensure you were accessing the correct fields. Today’s automation allows you to scroll through a list of needed fields, select the ones that are necessary for your report, move them around on the page and select ‘run.’ The application then accesses the necessary information and provides you with the results. The act of automation has reduced the time necessary to run the report (allowing you to run it as many times as necessary to see the information you are looking for in the format you desire) as well as eliminating the need for you to contact IT in order to either extract the data or create the report for you.
Previously, when running statistical analysis, it was necessary to understand the structure of the information and run countless point-to-point analyses to determine if there was a correlation between data points that could then be used from a predictive perspective. Algorithms were built by hand using specific coding instructions in order to get results. While there are several programming languages today that allow data scientists to continue to delve into deep analysis and run ML programs dependent on that analysis to make specific decisions on a shop floor (for example), it is also now possible for the average user to drag and drop any number of fields into an application to see what the correlation between the fields is with no support from either a statistician or IT. What is important to understand here is that the automation that is included within all these tools (reporting, analytics, AI/ML, etc.) becomes transparent to the end user. All of the work that needed to be coded or done ‘by hand’ is now done in the background through the interface with automation.
And, while we did mention the idea of incorporating Data Governance and the cleansing of data as part of our previous article, we would be remiss if we did not mention it again here as the use of automation in this area (verification on consumption) also decreases the data rejection rate and increases the overall quality, and value of the data. The implementation of data standards and governance provide the business rules that are used to cleanse the data and increase its validity to the business itself. Automation and the use of AI decrease human intervention in the cleansing process, increasing the velocity at which the data can be used.
In summary, organizations should embrace change and watch for opportunities to harness and leverage data to improve profitability, reduce costs and increase revenue. Advances in automation of the data lifecycle enhance our ability to acquire, store, cleanse, integrate and deliver data in real time – thus improving the overall value and reliability of the massive amount of information – and do so at the speed of thought.