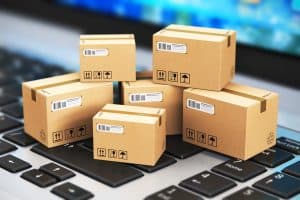
Automation will continue to grow and expand within logistics operations through the use of technologies such as artificial intelligence.
Automation uses technology to augment human effort across a myriad of tasks. In logistics, the potential for automation is massive, and the benefits are significant, especially when operations experience large variations or increases in demand. Scaling operations up typically requires additional staff who are often not immediately available, particularly during times when demand is also coming from other industries. Reacting quickly to market fluctuations requires fast action and additional capacity across the entire operation.
Logistics automation allows for rapid increases in capacity as demand changes. When used strategically, logistics automation increases productivity, reduces human error, and improves working efficiency. And with the right logistics automation software, hardware, and platforms resources in place, the impact on operational expenditures during periods of low demand are minimal and much lower than maintaining a large human workforce. As demand increases, the capacity is already in place and ready to be activated. While this gives logistics companies the flexibility needed to react quickly to changes in demand, there is the opportunity to do more.
See also: Logistics Market Needs Digital Transformation to Overcome Challenges
Artificial intelligence amplifies logistics automation impact
The introduction of artificial intelligence (AI) into logistics automation amplifies AI’s impact. AI reduces errors in common semi-skilled tasks such as sorting and categorizing products. Autonomous mobile robots (AMRs), for instance, improve package delivery, including the last mile of delivery which is typically the most expensive. AI helps AMRs with route planning and feature recognition, such as people, obstacles, delivery portals, and doorways.
Integrating logistics automation into any environment comes with challenges. It can be as simple as replacing a repetitive process with a powered conveyor or as complex as introducing a collaborative, autonomous robot into the workplace. When AI is added to this automation and integration process, the challenges become more complex, but the benefits also increase.
The effectiveness of individual automation elements increases as the solutions become more connected and more aware of all the other stages in the process. Putting AI closer to where the data is generated, and actions are taken, is referred to as edge AI. The adoption of edge AI is already redefining logistics automation.
Edge AI is developing rapidly, and its use is not restricted to logistics automation. The benefits of putting AI at the network edge have to be balanced with the availability of resources, such as power, the environmental operating conditions, the physical location, and the space available.
Inferencing at the edge
Edge computing brings computation and data closer together. In a traditional IoT application, most data is sent over a network to a (cloud) server, where the data is processed, and results are sent back to the edge of the network, such as at the physical piece of equipment. Cloud-only computing introduces latency, which is unacceptable in time-critical systems. One example where edge computing comes into play is capturing and processing the image data of a package locally during sorting enables the logistics automation system responds in as little as 0.2 seconds. Network latency in this part of the system would slow down the sorting process, but edge computing is removing that potential bottleneck.
While edge computing brings the computation closer to the data, adding AI to the edge makes the process more flexible and even less prone to error. Similarly, last-mile logistics relies heavily on humans, but this too is improved with AMRs using edge AI.
Adding AI has a significant impact on the hardware and software used in logistics automation, and there is an increasing number of potential solutions. Typically, the solutions used to train an AI model are not suitable for deploying the model at the network’s edge. The processing resources used for training are designed for servers, where resources such as power and memory are almost infinite. At the edge, power and memory are far from infinite.
The trend to heterogeneous architectures
In terms of hardware, large multicore processors are not well suited for edge AI applications. Instead, developers are turning to heterogeneous hardware solutions optimized for AI deployment at the edge. This includes CPUs and GPUs, of course, but it extends to application-specific integrated circuits (ASICs), microcontrollers (MCUs), and FPGAs. Some architectures, like GPUs, are good at parallel processing, while others, like CPUs, are better at sequential processing. Today, there is no single architecture that can really claim to provide the best solution for an AI application. The general trend is to configure systems using the hardware that offers the most optimal solution, rather than using multiple instances of the same architecture.
This trend points towards a heterogeneous architecture, where there are many different hardware processing solutions configured to work together, rather than a homogeneous architecture that uses multiple devices all based on the same processor. Being able to bring in the right solution for any given task, or consolidate multiple tasks on a specific device, provides greater scalability and the opportunity to optimize for performance per watt and/or per dollar.
Moving from a homogeneous system architecture to heterogeneous processing requires a large ecosystem of solutions and a proven capability to configure those solutions at the hardware and software level. That’s why it’s important to work with a vendor that has significant tier 1 partnerships with all the major silicon vendors, offering solutions for edge computing and working with them to develop systems that are scalable and flexible.
In addition, these solutions use general open-source technologies like Linux, as well as specialist technologies such as the robot operating system, ROS 2. In fact, there is a growing number of open-source resources being developed to support both logistics and edge AI. There is no single ‘right’ software solution from this point of view, and the same is also true for the hardware platform on which the software runs.
A modular approach to edge computing for automation
To increase flexibility and reduce vendor lock-in, one approach is to use modularization at the hardware level, making hardware configuration within any solution more flexible. In practice, modularization at the hardware level allows an engineer to change any part of the system’s hardware, such as a processor, without causing system-wide disruptions.
The ability to ‘upgrade’ an underlying platform (whether that be software, processors, etc.) is particularly important when deploying a new technology like edge AI. Every new generation of processor and module technology often provides a better power/performance balance for an inferencing engine operating at the network’s edge, so being able to take advantage of these performance and power gains quickly and with minimal disruption to the overall logistics automation system and edge AI hardware system design is a distinct advantage.
Modularization in the hardware is extended into the software by using a micro-service architecture and container technology like Docker. If a more optimal processor solution becomes available, even if it is from a different manufacturer, the software leveraging the processor is modularized and can be used in place of the module for the previous processor without changing the rest of the system. Software containers also provide a simple and robust way to add new features, which applies to running AI at the edge, for example.
The software inside of a container can also be modularized.
A modular and container approach to hardware and software minimizes vendor lock-in, meaning a solution is not tied to any one particular platform. It also increases the abstraction between platform and application, making it easier for end-users to develop their own applications that are not platform-dependent.
Conclusion
Deploying edge AI within logistics automation doesn’t require replacing entire systems. Start by assessing the workspace and identifying stages that can really benefit from AI-powered automation. The main objective is to increase efficiency while decreasing operation expenditure, particularly in response to increased demand during a time of labor shortages.
There is an increasing number of technology companies working on AI solutions, but often these are aimed at the cloud, not edge computing. At the edge, the conditions are very different, resources may be limited, and there may even be a need for a dedicated private communications network.
Automation will continue to grow and expand within logistics operations through the use of technologies such as AI. These system solutions need to be designed for use in harsh environments, very different from the cloud or data center. We address this using a modular approach that offers highly competitive solutions, short development cycles, and flexible platforms.