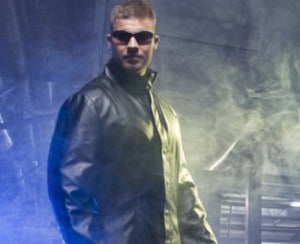
FICO’s chief analytics officer writes that as criminals start to learn our AI systems we must use defensive AI to thwart the bad guys.
I’m convinced we are entering the Golden Age of artificial intelligence (AI). With so much promise and potential in front of us, I am feeling a little like Neo in The Matrix as he swallows the red pill. (Alert: more Matrix references ahead.)
However, rather than science fiction, my recent work at FICO to make AI better has drawn upon my background in theoretical physics to create what we call defensive AI. I’m talking about the Heisenberg Uncertainty Principle — from the German physicist Werner Heisenberg, not Walter White — which states that “the more precisely the position of a particle is determined, the less precisely its momentum can be known, and vice versa.”
The Heisenberg Principle was an inspiration in my developing a Defensive AI method. Unfortunately, AI-based attacks are not science fiction — they are happening today.
Why we need defensive AI
Businesses have relied on AI to fight fraud and financial crime for more than 25 years. Before these neural network defenders came along, banks used rule-based systems to try to prevent fraud.
Back then, criminals were constantly testing these rules — if a $255 transaction at a grocery got stopped by the bank’s rules, would $245 be stopped? What about $199? Since the rules were static, fraudsters launched manual attacks, learning the rules and monitoring changes in the rules response, to understand how to maximize the amount that could be stolen “under the radar.”
Today, AI is commonly available and is considered “superhuman” at executing certain tasks, fraud detection being one of them. Criminals have had a harder time testing and understanding the complex inter-relationships between all the data elements that the anti-fraud neural networks combine.
But fraudsters, like New York City, never sleep. As business and society overall, become more and more dependent on AI, we must assume that criminals will want to reproduce the AI models. Once learned, criminals find the secret paths to commit fraud without detection, based on the unique behaviors of the AI system.
To find those paths, fraudsters are again testing and attacking, targeting the bank’s AI defenders with their own offensive AI systems. But now, instead of trying to figure out the thresholds that would trigger an anti-fraud rule set to stop the transaction — such as a new grocery store, for an amount greater than $250 dollars, between the hours of 3-6 p.m. — the attackers are trying to learn the AI model.
If the attackers can learn the AI defender and how it responds, they have the ability to anticipate its move, like a fight scene from The Matrix. The attacker will learn the model and determine what they can get away with. The fraudster could then run millions of transaction perturbations in a cloud testing environment, find those that look the most likely to succeed, and launch attacks based on expert, learned knowledge of the likely AI system.
We’ve long understood this risk. As such, defensive AI models incorporate adaptive components that make it harder for offensive AI systems to learn the neural network response.
How fraudsters create an AI attack model
Criminals can learn the AI we rely on by directing fake test data at banks’ AI systems. They could get access by pretending to be a new merchant, and/or by compromising a merchant system to gain access to a payments channel, as well as banks’ testing and governance partitions.
Criminals may even try to steal the AI models outright through cyber breaches. If stolen, these obfuscated and encrypted models may still respond to testing transactions.
Once they have access to the defender AI, fraudsters would likely send batches of testing transactions, millions at a time. The criminals would get a fraud score for each transaction and attempt to map out likely transaction sequences, monitoring the behaviors of the model. In other words, using AI of their own, criminals can create an AI model to produce the same score response to their testing. This is the offensive AI model, constrained by the quality and effectiveness of the criminals’ testing and the volume of testing transactions.
With their offensive AI tech nailed down, the criminals could steal expertly, working to circumvent the bank’s AI model response with unique transactions that the bank’s anti-fraud system might have not been seen before.
Banks that depend on anti-fraud AI systems need to keep them protected, and monitor data streams that may be pointed at the model to ensure that they are legitimate. But what can the AI do itself to prevent being learned? This is Defensive AI.
Defensive AI outsmarts criminals
Defensive AI models selectively deceive or return incorrect outputs if the models believe they are being monitored. They might return scores that are backwards, or create patterns that make the adversary modeling data set inaccurate and consequently the attacker’s AI less effective. Clever score responses could even guide the defensive AI to create artificial patterns in a learned offensive AI, making the criminal’s use of the offensive AI model easier for the bank to detect.
The defensive AI system could also bias the responses for the attacker AI to be misled, to generate data of a particular form. For example, the AI may decide it will give much reduced (non-fraud) scores to the attacker’s test data for electronic purchases between $300 and $500. Later, in production, the Defensive AI system can determine if the attacker took the bait, and then rapidly isolate the new transactions of this behavior and the sources, to turn over to law enforcement.
Just like Neo
Defensive AI thwarts criminals’ attempts to measure it. As such, criminals and their AI will find it much harder to determine which responses from defensive reactions are legitimate. The criminals will wonder, “Is it real, or is it The Matrix?”
This has ramifications not just for fraud but for defense and other areas. We should expect that AI-based attacks are going to happen across the spectrum. For example, if I wanted to infiltrate a maximum security institution that used facial recognition software, I would work to figure out which of my images might be best misclassified as a legitimate security member, or manipulate my face in such a way to mislead AI to determine close-enough match. This is why defensive AI is such an important area for research.
I’m going to catch up with my old friend Neo now on Netflix. Share your favorite Matrix moments on Twitter, where I’m @ScottZoldi, or in the comment section below.