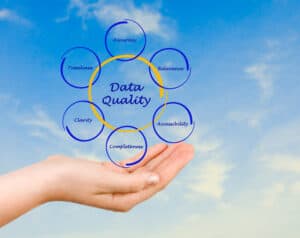
The quality of your data determines the success of your migration. So, part of the process is finding your outdated, irrelevant, and duplicated data so that you’re only migrating high-quality data that will provide business value.
Businesses wishing to migrate their data do so in the hopes of gaining immediate advantages. Among the primary business value-adds stakeholders seek to achieve with the implementation and migration of new systems are optimization and the unification of data under a single system. Yet many data migration projects fail. Why? The common denominator is almost always poor data quality.
When it comes to any sort of data migration project, data quality is critical. But why does it matter if you have poor-quality data? For one thing – it’s expensive. It’s estimated that poor-quality data costs organizations an average of $12.9 million a year.
The key to addressing this challenge is first understanding the difference between good and bad quality data – then, you need to focus on ensuring you’ve got high-quality data.
Poor quality vs. high-quality data
A recent study we conducted with HFS Research found that although 80% of respondents stated they trusted the data in their organization, less than half thought at least 60% of that data could be operationalized. In other words, less than 2/3 of the data that businesses gather is useful and consumable. Poor quality data is an extremely common problem – and one with real consequences, especially when it comes to conducting data migrations.
What does poor-quality data look like?
Irrelevant data: Let’s say you have 25 years of sales orders – you probably don’t need to clutter up your new system with all that data. You need to evaluate whether the data you have is still relevant – and only the relevant data should be moved. This will help you focus on what you need to think about during a migration.
Duplicative data: Duplicates represent truly bad data that you don’t want to port over. You will likely spend a lot of time looking at duplicates to make sure you don’t have two – or more – records of the same material, customers, or vendors.
Old/outdated data: You might have inventory records for materials that you haven’t bought, sold, or shipped in 10 years. You clearly don’t need to migrate that kind of data.
See also: Data Quality Remains Biggest Detriment to AI Success
Why high-quality data matters in a migration
In contrast, high-quality data is relevant data. You need to ensure you have the right set of data, the relevant data, as step Number One. Organizations all too frequently concentrate solely on moving data from one system to another when working on large-scale projects like data migrations instead of re-assessing their entire data management strategy. Otherwise, how can you be sure that you’re moving data in a way that matters to (and benefits) the company?
You don’t want to move a ton of data unnecessarily; doing so adds complexity and cost. Data quality problems are a major cause of migration project cost and schedule overruns.
See also: You’ve Migrated to the Cloud, Now What? 4 Critical Cost-Saving Practices
Best practices for success
First, you need a methodology to break the giant problem down into small, repeatable steps. Data migration is not a “throw it at the wall and see what sticks” kind of process. You need to be able to answer these questions:
- How do I amass the data?
- How do I document the requirements?
- How do you apply the transformation rules?
- How do you test the quality of the results?
- In what order do I load the data?
- What tools do I use to load it?
- How does the process I’ve created handle changes in design or requirements?
- How do I handle bad data?
All of these, and many more, are predictable steps of a data migration. Each step needs its own process. The collection of these smaller problems and solutions, done well, creates a repeatable methodology for successfully completing the larger, complicated process of data migration.
Second, understand that data migration is not just a one-and-done thing – it takes iterations and a lot of decisions along the way. The key here isn’t “knowing” what your data quality is already. It’s figuring out what your data quality is with regards to your new system – how to determine the quality of your existing data based on the new rules and requirements of your new target system.
Third, seek out proven expertise. Perfect cycle counts in your legacy inventory system are great. But how will the inventory work in the new Enterprise Warehouse Management system that has many new rules, new requirements, and new processes that your old system didn’t have? Most likely, there are requirements and features in your new system that your legacy data can’t support yet. A partner with proven expertise will help you find those and help you figure out what to do next.
Quality determines success
The quality of your data determines the success of your migration; there are no two ways about it. So, part of the process is finding your outdated, irrelevant, and duplicated data so that you’re only migrating high-quality data that will provide business value. Check your process against the best practices discussed above to make sure you’ve got the best chance possible of executing a meaningful, valuable data migration.