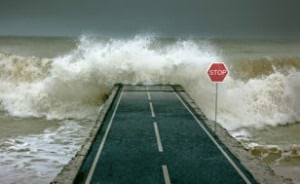
Is predicting the weather simply too complex a problem for current AI technology and computational power?
The stories coming out of Texas have been harrowing, to say the least. The sheer scale of flooding from Harvey is catastrophic, with bayous in the Houston area at 500-year levels. The rain might be passed in many areas, but the fallout from widespread, severe flooding remains.
From hundreds of miles away in Arizona, I nervously watched water levels in the bayou closest to my Houston family. Between that, and as someone who writes about artificial intelligence (AI), advanced analytics and big data on a regular basis, I started to wonder about why we haven’t heard more about the growing use of AI in weather forecasting and storm preparedness. It seems like a highly valuable target — after all, according to The Washington Post, this is Houston’s third 500-year scale flood in the last three years. Any improved preparedness could have enormously positive effects.
[ Related: How AI Can Aid the Agriculture Sector ]
The truth is that, in many cases, weather might simply by too complex a problem for our current AI technology and computational power. That’s not to mean that researchers aren’t trying, however. Predicting the inherently unpredictable comes with enormous challenges and slow progress, despite of the hard work of many.
Making weather easier to understand
Sophisticated statistical analysis and complex algorithms are excellent tools for professionals, but do very little for ordinary people. Perhaps, if weather were presented in easier and more accessible formats, the general public could follow along more reliably. That’s the idea behind a new technology from AccuWeather, which “handles plain-language questions from users and provides easy weather answers and localized forecasts.”
The system is available on Facebook Messenger, which makes it easily accessible, and can handle tricky questions, such as whether someone should bring an umbrella with them on a given day. This system is unlikely to be helpful in a Harvey-like event right now, but these kinds of system tend to get better with time, and more interactions with users.
[ Related: How AI and Cognitive Science Can Beat Addiction Treatment Fraud ]
Back in 2015, IBM bought The Weather Company, parent to the Weather Channel and Weather.com, with the intention of rolling out a similar natural language processing service to Watson. But there has been much news about it since, proving that IBM is trying to do things quietly, or that using AI to predict the weather is still very much a work-in-progress.
Can AI even do forecasting?
Even an AI optimist would be lying if he said they could plot a date where AI overtakes the current forecasting methods. We’ve been putting our best meteorological brains (supercomputers included) toward these problems for decades, and forecasting is still incredibly difficult. It’s not that these researchers are bad at their jobs, it’s that there’s just too many inputs and variables to keep track of and account for. The progress that’s been made is nothing short of miraculous.
But, on a fundamental level, AI might not be the right tools for the job — at least for the foreseeable future. A deep learning model improves itself by looking at its set of data for specific variables. In contrast, with a self-driving car obvious variable are signs, other vehicles, pedestrians and painted lane markings. Have a self-driving deep learning algorithm “watch” enough dashcam footage for these variables, and you’ll eventually find your way to a system that knows how to read and avoid these features. It won’t be easy, but many companies are proving it’s entirely possible.
[ Related: Why the U.S. Military Is Gung-Ho About Autonomous Boats ]
Given that weather is entirely defined by physics, it’s theoretically possible to create a deep learning model that can account for every variable. If you dive deep enough, you’re essentially trying to teach your AI the entire science of fluid dynamics. Even with all our supercomputers, that’s just not a viable way to model weather in the days or weeks to come. On top of this, weather is a classic example of a system affected by chaos theory, in which small changes to the system creates massive, and oftentimes random-looking, consequences.
The high bar to AI forecasting
Deep learning and AI has a small place within forecasting, but nothing has taken over the existing physical models. The Weather Research and Forecasting (WRF) Model purports to be using some deep learning-esque techniques in its analysis, and small research groups are experimenting with using machine learning to predict future rainfall intensity in a small region over the next hour or two.
But instead of trying to use AI to predict the future, there is promise in using the technology to look into the past. The weather industry has decades of modeled forecasts and observed weather data, which could be analyzed by deep learning algorithms. If failed forecasts can be cross-referenced to the observed reality, perhaps this AI could discover faults in existing models, or reveal previously-unknown opportunities.
Whether that would ever result in a better response to such a monumental hurricane as Harvey is still unknown. Even a complex deep learning problem like self-driving cars has the advantage of having to worry about only a few habitats: roads, driveways, and parking lots. Meteorologists must worry about everything. If the industry shifts in that direction, it will likely be the most complex problem deep learning has been asked to solve yet, but the benefits could be truly incredible.