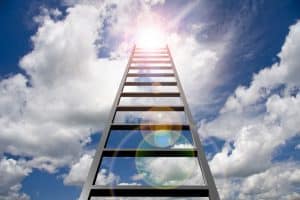
Businesses that work up the AI ladder will eventually be able to reach the top rung and have pervasive AI throughout their business processes
Continuous intelligence (CI) delivers maximum benefits when artificial intelligence (AI) is infused into all business processes. But how close are businesses to achieving this level of pervasive AI? And what are the consequences when it is not?
A core pillar of ascending what IBM calls the AI ladder is to use the technology to automate processes and scale across all processes. Only then will businesses reap the full benefits AI offers for improving the efficiency and productivity of teams across a business, whether automating routine tasks for sales and service teams or optimizing processes to achieve greater customer lifetime value. Ultimate benefits come when the use of AI executes on its own, without human intervention. With automation, there is no delay waiting for someone to locate the required data, put it into the right format, and run the most suitable algorithms for the problem at hand. As AI becomes part of all business processes, any solution must offer scalable performance that can adjust the processing resources based on the task at hand.
See also: The Case for Continuous Intelligence
So, where do most companies stand on the rungs of the AI ladder? As one would expect, AI adoption is not consistent across all companies. Analysts say they are seeing a new digital divide, a divide between the AI haves and the AI have-nots.
In 2020, says Forrester, the “tech elite” will ramp up AI plus design skills while another will “fumble.” Pairing human-centered design skills and AI development capabilities will be key. As for the rest of the workforce, by 2024, 75% of enterprises will invest in employee retraining and development, including third-party services, to address new skill needs and ways of working resulting from AI adoption, predicts IDC.
Also, in 2020, Forrester predicts that 25% of the Fortune 500 will add AI building blocks (e.g., text analytics and machine learning) to their Robotic Process Automation (RPA) efforts. Such activities correspond to a different step of the AI ladder. Specifically, such adoption is part of the “organize” rung of the ladder. Businesses that reach this rung essentially create a trusted analytics foundation.
Such a foundation is needed because most business managers do not understand AI. Worse, many organizations lack the data science skills required to implement, develop, and deploy modern analytics solutions. A trusted analytics foundation can overcome these limitations by offering the necessary tools, algorithms, and engines to make it easier to conduct advanced analytics. Key elements of a trusted analytics foundation should help simplify model development, as well as make use of proven industry-specific AI algorithms. Such an approach makes it easier for businesses to deploy custom CI solutions.
Just to round out the picture, the other two steps of the AI ladder include:
- Collect: Make data easily accessible for use by analytics applications. Businesses can accomplish this by adopting a data and information infrastructure that enables self-service data-prep and ingestion capabilities. Why is this capability needed? Any CI solution or efforts to infuse AI into business processes will not work or be successful if users have difficulty incorporating new sources of streaming data. AI applications are only going to be as good as the data they use.
- Analyze: Scale insights with ML everywhere. ML applied to streaming data delivers insights that help businesses better understand and cater to their customers, make more strategic business decisions, and optimize company workflows. As such, smart businesses must deploy ML across all operational areas to reap maximum benefits. Fortunately, the convergence of easy access to inexpensive cloud computing and solutions that simplify the integration of large data sets provide the infrastructure that enables ML everywhere.
- Trust: Achieve trust and transparency in outcomes. The analytics used in many AI efforts are black boxes. There is little transparency as to how the algorithms and models work or derive actionable intelligence from the data. At a minimum, this lack of understanding about the analytics can lead to erroneous conclusions. For example, if a healthcare ML model developed to diagnose a condition using lab results and a patient’s medical history only uses a small segment of the population to train the model, the model’s conclusions when run for patients outside that narrow population may not be valid. Another emerging issue is that an ML model might be biased because it uses erroneous assumptions, inherent prejudices, or a limited set of data to train the learning algorithms. The solution: AI should not be a black box; business professionals need justification mechanisms that support conclusions. In this manner, businesses will be able to trust the recommendations made by AI software.
Businesses that work their way through the steps of the AI ladder will eventually be able to reach the top rung and have pervasive AI throughout their business processes. In this way, there will be no AI haves and have-nots. AI will be accessible to everyone in the organization.