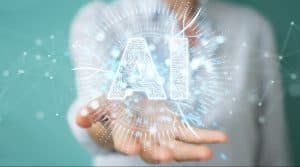
As high-profile bias cases surface, companies must review their own AI-initiatives for even low levels of bias or risk losing valuable consumer trust.
AI has a bias problem; no arguments. It’s a well-noted fact. Reducing AI bias is critical if businesses want to survive the great extinction event of data-centric business models.
It’s not just a moral quandary, though that’s reason enough all on its own to create transparent and responsible AI-driven initiatives that cover a range of use cases without adding to the problems it’s trying to solve. Businesses that rely on biased AI could lose reputation, trustability, and competitive edge.
See also: FICO’s Scott Zoldi Talks Data Scientist Cowboys and Responsible AI
Why AI bias Matters
Well known examples of AI bias — from Microsoft’s failed experiment with twitter to the failure of autonomous vehicles to recognize Black pedestrians as people — are embarrassing and dangerous. Their high-profile nature causes some businesses to believe that their minor bias is just an inconvenience. Hiring bias doesn’t kill people or harass them on social media, so what’s the big issue?
Even minor bias can chip away at a reputation in a climate that demands attentiveness online (always) and, at the same time, distrusts that same attention. Businesses that slip up using artificial intelligence initiatives will be hard-pressed to win back trust over even smaller breaches.
The most common business use cases, such as deep learning, could account for potential billions in value if deployed correctly. Businesses already worry about adoption, with many leaders admitting that while they believe AI is critical to success, they haven’t taken full advantage. Add a layer of bias on top of that hesitancy, and businesses could lose out big in the coming years:
- misreading data for disastrous marketing results
- losing out on top talent because of AI-driven hiring practices
- missteps in performance reviews and employee culture
These are just some examples we know. Bias can leak into every part of business operation because AI and data-driven initiatives are integral parts of those same operations. When you deploy full adoption without examination, that bias is now part of your entire organization.
What researchers are doing to reduce the problem
A lot of ink has gone into the discussion of “data versus algorithm,” but reducing bias is a multi-step approach. Initiatives must address the data that goes in and the conclusions that come out through transparent and fully vettable systems. While this won’t happen overnight, companies should consider a three-step approach to deploying non-biased AI.
Address training data
We know the garbage-in-garbage-out theory, but it’s not always bad data that causes bias. Sometimes, small inconsistencies in training data or gaps in exposure. For example, when Amazon created an internal hiring tool, the algorithm showed discrimination against women. How did this happen?
The training data looked at successful hirings from the past years. Still, in the tech field, covert bias and lack of diversity inadvertently taught the machine to prefer male candidates over female ones, going so far as to eliminate resumes based on the presence of all-female colleges in education sections.
The answer here is careful attention to the training data and the results. Noticing a lack of diversity or an overwhelming preference for a certain type of candidate takes a trained eye, but this is an important step.
In-processing models
AI can also participate in bias-reducing activities during training and processing. For example, adversarial models can run concurrently, minimizing errors for the primary objective while a second model penalizes the first for using certain parameters.
Adversarial models got their start in cybersecurity, where one model actively tried to break into another. In anti-bias, the models are trained to check each other as they work through a task such as hiring recommendations.
Post-processing models
The final piece to this puzzle is a safeguard in place after the training and processing complete. These principles examine the results of any given process and identify potential pitfalls, such as an overabundance of certain criteria (i.e., gender-based recommendations) or automatic review when the model is least certain of an outcome.
By safeguarding the conclusion, companies have the chance to review recommendations and conclusions. Suitable training helps ensure that all conclusions have a level of transparency that allows for review and pivoting throughout AI use.
Putting it all together
Consumers still aren’t fully invested in the use of artificial intelligence, putting the onus of trust onto companies. As more high-profile bias cases surface, companies must find a way to review their own AI-initiatives for even low levels of bias or risk losing valuable consumer trust.
Companies must be able to capture data to make informed decisions, but data-capture isn’t going to be easy without consumer trust. As internet and data-savvy consumers become more prevalent, businesses will have to prove that their data and artificial intelligence initiatives are 1.) transparent and 2.) free of bias.
The three-step system of checking behind artificial intelligence conclusions allows businesses to create true value that doesn’t leave money on the table — in concrete terms (fines or lost revenue) and soft cost (losing out on top talent).
We cannot create truly unbiased AI because humans are not unbiased. However, this doesn’t mean companies can rest on current systems. AI will become a differentiator to business success. To wrap up:
Fairer AI is possible
- Training samples must include a variety of diverse criteria
- A diverse team helps reduce overall bias and spot covert bias in training data
- Regular audits are a must, both for training data and in conclusions
- Models that continually check during in-processing reduce further bias potential
- Anti-bias work in AI is not a suggestion — it’s a necessity.