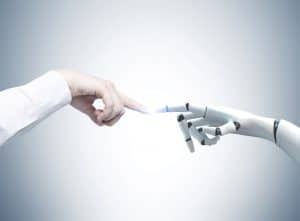
Data quality. Integration. Governance. DIG?
Over the next several years, both AI and machine learning (ML) software will be essential for businesses to stay competitive, enabling targeted customer interactions in both B2B and B2C settings while bolstering operational efficiencies.
Market research firm Trifactica predicts the global artificial intelligence software market will experience massive growth in the coming year, increasing revenues from around $9.5 billion in 2018 to a projected $118.6 billion by 2025.
See also: Not Good at Analytics? Not Ready for AI
One major factor contributing to this explosive market growth is the increase in research and development of AI and ML technologies. In fact, R&D is so widespread that’s is hard for companies to keep up with key disciplines that provide the necessary underpinnings of AI and ML use cases – namely data quality, data integration, and governance.
Unless significant progress is made in these three areas, businesses implementing AI and ML will have a difficult time achieving their ultimate vision—fully automated, accurate and smart businesses that are uniformly compliant across the enterprise, occurring in real-time with minimal human intervention. For example:
Data Quality: AI and ML initiatives, and the critical business and customer-facing decisions they support, are only as strong as the quality of the data feeding the algorithm. High-quality data—generally considered to be data that is consistent, trustworthy, accurate and complete—is essential in an era of automated decision-making.
While there are a variety of tools available on the market, poor data quality continues to run rampant in many enterprises.
“Dirty data” can result from a variety of causes, including manual data entry errors, optical character recognition (OCR) mistakes, data transformation errors, duplicate data and more. The costs of poor data quality can be severe in terms of reputation. There are countless examples of data quality fails – one retailer made the embarrassing mistake of sending a free personal care product designed for 18-year-old men to their broader customer base. According to Forrester Research, nearly one-third of data analysts spend more than 40 percent of their time vetting and validating data before it can be used for strategic decision-making.
To render data as agile as possible, organizations must be ready to use it in real-time, meaning they must have full confidence and trust in the data without falling into “paralysis by analysis.” In fact, human intervention should only be allowed when the potential business impact of taking the wrong action or making a mistake is relatively minor; the default for any major decision should be to the AI system as opposed to human instinct. As an industry, we are not quite there yet.
Data Integration: AI algorithms work best when they are based on the richest, most comprehensive information. Consider popular mapping applications where the more high-quality, accurate data is represented (street names, traffic light locations, buildings, and landmarks), the easier and more intuitive it becomes to reach the destination.
In a customer relationship management (CRM) scenario, for example, a customer can send a message through a company’s website support widget. An AI tool enabled with text processing then deciphers the tone of the message. If it is classified as negative, a support staff member can be notified and assigned. The CRM system is also accessed to review the customer’s history, which provides context to support the customer interaction.
AI algorithms cannot work well when enterprise information is siloed. However, recent surveys show that integration hurdles are prevalent, with more than half of all data scientists devoting most of their time to integration-related tasks. The strongest AI applications depend on rich data lakes that pull and integrate data from disparate sources—the cloud, back-end legacy systems, ERP systems and databases among them. This can be a lot harder than one might imagine given that all data must be cleaned, accessible and compatible.
Governance: One of the great things about AI algorithms is that once a proof of concept has been established in one department, the algorithm can be used elsewhere by making modifications to meet that department’s unique needs. What is often harder to achieve, and tends to not be so uniform, is enterprise-wide AI governance—that is, ensuring that AI is only used in certain ways that align with the ethical principles and values of the organization.
It can be difficult to enforce a uniform approach to governance across all departments, given that different enterprises have articulated different sets of AI principles. Microsoft, for instance, includes a call for transparency (AI systems should be understandable) and accountability (it is possible to track back the algorithm to explain certain outcomes, for example, why a person was denied a credit card or why a request for medical treatment was rejected).
AI and ML have the potential to touch peoples’ lives in very personal ways, making it imperative to enforce enterprise-wide rules ensuring AI systems are designed, developed and deployed in a manner that maintains accuracy, fairness, ethics, data privacy, and security. Effective AI governance is something that many organizations struggle with. But as AI adoption increases, effective governance will be critical to ensuring the perils of AI do not outweigh the promise.
Needed: Clear strategy and purpose
With AI and ML technology developing at such a rapid pace, it can be hard for businesses to keep up in ancillary areas, namely data quality, data integration, and governance. As business decisions are increasingly machine-driven and the role of human intuition diminishes, it will be critical to address these foundations.
It takes a clear strategy and statement of purpose to effectively implement sustainable AI practices. Once the vision is clear, culture needs to be addressed to create a matrixed organization of data owners across relevant lines of business and operational groups. Once this is established, awareness campaigns can motivate broader use of AI that will exponentially improve data quality, accessibility and governance. Just like the algorithm, the business begins to train itself. With that, AI becomes embedded in the operational culture of the company.