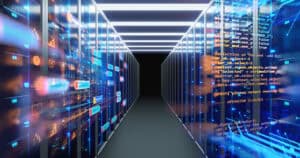
Unified storage that can easily work with multiple data formats, structures, and access mechanisms across a hybrid multi-cloud landscape provides a strong foundation to achieve these goals and accelerate AI workflows for both predictive and generative AI.
Since AI really took off five or six years ago, it’s become one of the greatest technological disruptors ever seen. Enterprises are increasingly using AI across multiple areas of their business to deliver critical insights, operational improvements, increased customer satisfaction, and better products/services. Needing to work with a variety of data formats (i.e., image, video, text, audio), data structures (i.e., structured, unstructured, or semi-structured), data access mechanisms (i.e., block, file, object), and data locations (cloud, on-premises, edge), many still struggle with the data management necessary to enable AI and make magic happen.
This challenge is in no small part due to the difficulty in unlocking data silos across the organizations. And business leaders are increasingly impatient, looking for either a first-mover advantage or, more recently, just to keep up with their competition. Accelerating the data science and engineering work that drives AI starts with the right data architecture and storage, ensuring data becomes more accessible, and data science workflows can proceed faster. The enterprise has the data, but ensuring data scientists, engineers, and IT can work together optimally is the key to more rapidly realizing the business potential of AI.
IT plays a critical role in making AI real
To ensure data scientists are working efficiently, the pressure is on IT to enable data architectures that seamlessly move and manage multiple data formats, data structures, and data access mechanisms so a wide variety of data can be quickly incorporated into the AI workflows built by data engineers and used by data scientists. IT must adopt flexible, unified storage characterized by a joined-up operating environment for managing any data type (structured or unstructured), wherever it’s needed, on-premises, or across every major public cloud.
AI has made the complex job of managing an increasing number of data types residing in bespoke data silos exponentially more critical than ever before. IT needs to make access to the data simple so that data scientists and developers can do their work without having to care about the infrastructure that is making it happen, enabling data engineers to be as productive and effective as possible. A data scientist isn’t thinking about whether their data lives in a unified, intelligent data architecture or whether data sits in an object store or a file share, but they will absolutely notice and experience frustration if data is not all readily available, wherever they might need it—quickly and fully secured.
Data architectures that support AI development and run-time must operate at scale and support all data, no matter where it’s located, throughout its lifecycle, and maintain optimal flexibility and security every step of the way. The more simplified the experience is for the users tasked with developing the AI and driving business innovation, the more successful projects will be without worrying about the very real complexities that exist behind the scenes.
See also: Harnessing Real-time Data: Transforming Data Management with Artificial Intelligence
The role of data infrastructure in leveraging foundational models
Simplifying data access and speeding time to value are important in predictive AI and, equally, in the application of generative AI to enterprise use cases. Foundational models, ML models trained on a broad spectrum of generalized and unlabeled data and capable of performing a wide variety of general tasks such as understanding language, generating text and images, and conversing in natural language (e.g., GPT-4, BEBERT, LLama 2) are the starting point for using Generative AI in the enterprise. However, developing foundational models in-house requires considerable investment in expertise and computational power. Very few enterprises have the financial resources or time for the massive investments required to train their own.
Fortunately, it’s not necessary to do this. Companies can use their own data to augment existing, open-source foundational models using techniques such as retrieval-augmented generation (RAG). This has been a game changer because it means enterprises don’t have to do AI development from scratch. They can access and use foundational models already available in any of the major public clouds, available as a service, or free-to-use models that can be implemented on-premises.
These models have been trained on massive amounts of public data. However, they must still have the context of a company’s proprietary data to deliver value to a specific company use case. Whether this is through fine-tuning a Generative AI model or leveraging techniques like RAG–on-premises or in the cloud–the ability to feed in data quickly, easily, and securely from across the enterprise will be the difference between success and frustration in implementing a variety of productivity and revenue-generating enterprise use cases. The right data architecture and its underlying, unified storage infrastructure can simplify the whole process.
Choose unified data storage to accelerate AI workflows
Businesses that can simplify access to data from all sources are those that will be most successful and realize faster time to value with their AI initiatives. IT must create a flexible data architecture that allows data scientists, data engineers, developers, and others across business lines to bring together, prepare, manage, and use datasets with simplicity so they can focus on the data science rather than where the data is generated, lives, or how it’s stored. Unified storage that can easily work with multiple data formats, structures, and access mechanisms across a hybrid multi-cloud landscape provides a strong foundation to achieve these goals and accelerate AI workflows for both predictive and generative AI.