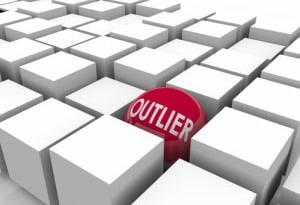
Anodot outlines a machine learning-based approach to anomaly detection with time-series data.
A few weeks ago, an Amazon Alexa-related issue cascaded from one house to many, but according to one company, it was entirely avoidable. And while this incident was benign and easily resolved, the issue itself is indicative of a bigger problem: How do we detect and recognize when our AI-controlled systems get something wrong?
The incident in question began when 6-year-old Brooke asked her family’s Alexa device a rather simple question: “Can you play dollhouse with me and get me a dollhouse?” In doing its level best to offer a great experience, the AI hooked in with Amazon’s purchasing services and bought a $170 dollhouse, plus four pounds of cookies. The family was able to return the dollhouse—Amazon offers free returns on “accidental” orders—and decided to keep the cookies.
The situation escalated when San Diego’s XETV-TDT aired a short report about the incident, and the anchor repeated the key phrase: “Alexa order me a dollhouse.” The station was immediately flooded with complaints from consumers their own Alexa devices tried to order additional dollhouses. It’s this kind of difficult, anomalous behavior that most AI systems aren’t quite capable of dealing with yet.
In defense of Amazon and its Alexa devices, owners can protect themselves with a confirmation code or turn off the feature entirely, and a little up-front work from the customers could have stopped the chain reaction in its tracks.
Even then, Rebecca Herson, the vice president of marketing at Anodot, says this situation was not only entirely avoidable, but should be a warning to companies making real-time business decisions based on streaming data—there need to be systems in place to detect when something out of place happens, and let the right people know. Anodot brands itself as a “real-time analytics and automated anomaly detection system” that takes advantage of machine learning to isolate issues and make those rapid-fire decisions safer and more dependable.
Time series anomaly detection
Herson says that if Amazon had been empowered by Anodot technology in this case, “Anodot would have flagged the dramatic spike in search terms and placed dollhouse orders and alerted the responsible Amazon team to the issue for further investigation.”
This is made possible by the backbone of Anodot’s system, which is a combination of internally-created and existing machine-learning algorithms that are upscaled to handle vast quantities of time series data in real time. In this scenario, Anodot would have been crunching Amazon’s time series data to determine the normal range for dollhouse searches and orders. When the spike in Alexa-powered purchases rose beyond normal limits, Anodot would have triggered a response and applied a significance score to help Amazon determine how fast and comprehensive the response might have been.
She adds: “Our machine learning system gets smarter with more data from a company, creating the best kind of symbiosis for our customers—more data makes their analysis smarter.”
This means that anomaly detection could be useful for many more applications, such as Fintech, IoT, online gaming, and digital publishing applications as well. For IoT, anomaly detection can be used to monitor the average performance of similar devices to determine which might be in need of maintenance or replacement.
On top of that, aggregating and analyzing data this way means that companies can help root out and uncover issues or risks—positive or negative—that they might have otherwise missed.
Herson says these kinds of unexpected insights, delivered via an easy-to-use interface, are one of the next steps companies can take to learn more from their existing data: “Business analysis practices and delivery has been stagnant for years, and there has always been a negative correlation between analytical ability and an organization’s decision makers who have the authority to react based on business intelligence. The benefits of seeing every metric laid out is immense—not only do users see issues and find root causes faster, they are able to correlate normally disconnected data to discover new opportunities for growth.”
We’ve seen before the tactic of using historical data to better understand streaming data, or ecommerce companies creating recommendation engines, or using real-time analytics with IoT for preventative maintenance. Real-time analytics isn’t exactly new, but the particular angle—discovering anomalies as they happen, not once customer service gets slammed with new calls and emails—could present new opportunities for a variety of industries.
As requirements get faster and more data moves toward the “edge,” finding anomalies might just might present the way for companies to discover issues not on the fringes, but also at the core of their business.