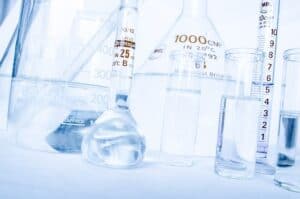
A data-driven approach to Pharmacovigilance holds immense promise and will pave the way for a more informed and holistic evaluation of drug safety, ultimately leading to safer and more effective treatments.
Emerging intelligence capabilities are significantly transforming the healthcare and life sciences industries. Specifically, the field of pharmacovigilance (PV), which is dedicated to monitoring drug safety, is undergoing a paradigm shift driven by the need to improve adverse event (AE) identification. Recognizing and addressing these challenges requires the examination of new patient reporting channels and the adoption of novel technologies, such as artificial intelligence (AI) and natural language processing (NLP), to better interpret, extract, and analyze patient feedback.
Challenges of Current Pharmacovigilance Processes
Current PV reporting systems primarily rely on structured data capture methods, including direct AE reporting by healthcare professionals (HCPs) and data collection from patient registries and regulatory databases. However, this approach has limitations. These traditional reporting methods, often involving manual processes and fragmented systems, may not fully capture the complexity of drug safety events, potentially leading to limited patient safety insights.
These methods frequently result in underreporting and incomplete data collection. Studies within the life sciences industry consistently report limited visibility of adverse drug reactions (ADRs). Regulatory bodies, such as the Food and Drug Administration, estimate that their Adverse Event Reporting Systems capture only a fraction of all ADRs, potentially between 1% and 10%. This significant underestimation of ADRS underscores the need for more innovative PV strategies to gain a more comprehensive understanding of drug safety risks.
New Channels for Patient Safety Reporting
As the industry acknowledges the chronic underreporting of AEs, organizations are examining new channels to collect and monitor patient safety signals. Empowered by online platforms, particularly social media, patients are actively sharing their experiences and seeking health information online. A recent study found that 85% of patients utilize social media for health information. Recognizing this shift, clinical research professionals are actively exploring new strategies for gathering patient feedback.
Unlike traditional methods reliant on structured data from registries and databases, online channels offer a new avenue to collect unstructured patient feedback. Social media platforms, online forums, and discussion groups provide a rich source of real-world patient experiences. This data contains valuable and authentic details about potential AEs.
Similarly, patient support programs (PSPs) often collect real-world data (RWD) that can complement traditional clinical trial data. This RWD provides invaluable insights into the safety and effectiveness of treatments in diverse patient populations and under varied conditions. Combining HCP reports with patient-reported data from PSPs contributes to a more comprehensive safety profile for pharmaceutical products, leading to a more thorough understanding of treatment performance and safety.
However, extracting meaningful insights from online patient safety data presents a unique challenge. Unlike traditional databases with standardized formats, social media conversations and PSPs are inherently fluid and lack uniformity. Inconsistencies, errors, and occasional absent information can pose hurdles in interpreting and relying on this data for PV purposes. The ubiquitous presence of emojis, slang, and colloquialisms complicates the process of identifying potential AEs.
See also: Beyond Lab Coats and Traditional Alchemy: Redefining Pharma R&D with AI/ML and Advanced Analytics
Use of Intelligence Eases Unstructured Data Extraction
Fortunately, advancements in technology empower PV teams to navigate this challenge. By leveraging the power of AI and NLP, PV teams can analyze and organize this vast amount of unstructured data. This transformation allows them to convert online patient narratives into a valuable resource for patient safety monitoring.
Organizations can leverage these technologies by developing algorithms and identifying specific terms or patterns within patient data. This allows for the automated detection of potential AEs from unstructured sources like social media conversations. Additionally, NLP bridges the gap between technical medical terminology and the language used by patients to describe their experiences. By analyzing patterns and word proximity within patient data, NLP-based models can facilitate the automated classification of AEs, significantly improving efficiency and accuracy.
Emerging research exploring the use of AI and NLP in PV has yielded promising results. For instance, one study analyzing a chatbot database successfully identified 78% of AE data from a massive dataset exceeding 292,000 virtual agent messages. The technology’s ability to process a vast volume of unstructured data and extract safety signals from a previously untapped source signifies the advanced capabilities of this technology. This holds immense promise for the future of PV, as it opens doors to gleaning valuable insights from a wider range of patient experiences.
Similarly, the use of AI, NLP, and optical character recognition technologies has streamlined the review of PSP data, reducing reliance on manual review. Studies have shown promising results, demonstrating the ability to achieve up to 90% efficiency, requiring human review for only a small fraction of records. This rapid analysis enables timely identification of potential safety signals, AEs, and emerging trends within PSP data.
Advantages of Intelligence in Pharmacovigilance Processes
These examples underscore the significant advantages of AI and NLP in expediting safety signal detection from unstructured data. Implementing these technologies can greatly improve safety event detection strategies and reduce the time needed to gather patient safety information. With these advanced analytical tools, clinical trial stakeholders can unlock the vast potential of unstructured patient data gleaned from online platforms and social media. This data-driven approach holds immense promise for the future of PV, paving the way for a more informed and holistic evaluation of drug safety, ultimately leading to safer and more effective treatments.