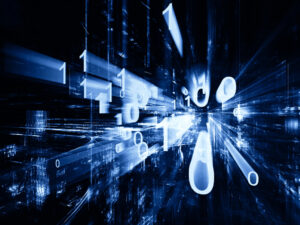
As data continues to play an increasingly central role in business operations and decision-making, the importance of efficient, flexible, and scalable data architectures will only grow. The integration of lakehouse formats with streaming infrastructure represents a significant step forward in meeting these evolving needs.
Data may be the oil of the 21st century in the way it’s driving innovation, competition, and organizational success, but that doesn’t mean it comes without challenges. More specifically, a rising gap is forming between operational and analytical data domains. Traditionally these two realms have existed in silos: While operational data focuses on transaction-heavy systems like SaaS applications and ERPs, analytical data resides in data warehouses and batch processing platforms.
This divide is rapidly becoming obsolete thanks to the introduction of Hybrid Transactional/Analytical Processing (HTAP) architecture which was introduced by Gartner in 2014, marking a significant shift in how we approach data management. HTAP blends operational and analytical data processing, enabling real-time decision-making and more agile business operations. While HTAP was initially associated with database systems, its principles are now being adopted across the modern data stack. This evolution has (thankfully) given rise to a new paradigm: the integration of lakehouse formats into data streaming infrastructure.
Lakehouse formats represent a convergence of data lake and data warehouse technologies, offering a unified approach to data storage and processing. When combined with streaming infrastructure, they create a powerful ecosystem that handles both real-time operational data and complex analytical workloads.
The importance of lakehouse formats in data streaming infrastructure cannot be overstated, and I’d like to explain key reasons why this integration is becoming crucial for organizations looking to stay competitive in the data-driven landscape.
See also: Data Streaming’s Importance in AI Applications
Unified Storage and Processing
One of the primary advantages of incorporating lakehouse formats into streaming infrastructure is the ability to achieve unified storage and processing. Lakehouses combine the scalability and flexibility of data lakes with the performance and structure of data warehouses.
This unified approach allows organizations to store vast amounts of raw data in its native format while still maintaining the ability to perform analytics on the real-time data. For streaming data, this means that incoming real-time information can be immediately stored and made available for analysis without the need for complex Extract-Transform-Load (ETL) processes or data movement between systems. The result is a more streamlined data architecture that reduces latency (i.e., delay) and improves overall system performance. Data scientists and analysts can work with fresh, real-time data alongside historical information, all within the same environment.
See also: Four Kinds of Software to Process Streaming Data in Real Time
Real-Time Analytics and AI Applications
In today’s fast-paced business environment, the ability to make decisions based on up-to-the-minute data is crucial. Lakehouse formats integrated with streaming infrastructure accelerate real-time analytics by ensuring that data is immediately available for processing as it flows into the system. This capability is particularly important for AI and machine learning (ML) applications, which rely on fresh, accurate data to make predictions and drive automated decision-making processes. By eliminating the delay between data generation and availability for analysis, organizations can create more responsive and accurate AI models.
For example, a retail company using this integrated approach could analyze customer behavior in real time, adjusting product recommendations and pricing strategies on the fly. Similarly, a manufacturing firm could use streaming data from IoT sensors to predict equipment failures and schedule maintenance proactively, minimizing downtime and improving efficiency.
Cost Efficiency and Simplified Management
Implementing lakehouse formats in streaming infrastructure leads to significant cost savings and simplified data management. Traditional data architectures often require multiple systems and teams to handle different types of data and workloads, often resulting in data duplication and increased complexity. By consolidating storage and processing into a single system, lakehouses reduce the need for data movement and replication. This not only saves on storage costs but also minimizes the risk of data inconsistencies and errors that can occur when maintaining multiple copies of the same information.
The unified nature of lakehouse architectures simplifies data governance and security. With a single source of truth for both operational and analytical data, organizations can more easily implement consistent access controls, auditing, and compliance measures across their entire data estate.
Flexibility and Scalability for Modern Data Needs
It’s no secret that the volume, variety, and velocity of data continues to grow exponentially, putting pressure on traditional data infrastructure. Lakehouse formats integrated with streaming systems offer the flexibility and scalability needed to handle these modern data challenges.
Modern data comes from multiple sources in varied formats, such as structured, semi-structured, and unstructured data. Most data scientists spend the majority of their time updating those data in a single format. However, when your streaming infrastructure has data processing capabilities, you can update the formats of that data at the ingestion layer and land the data in the standardized format you want to analyze.
Streaming infrastructure should also scale seamlessly like Lakehouse architectures, allowing organizations to add storage and compute resources as needed. This scalability ensures that the system can handle growing data volumes and increasing analytical demands without major overhauls or disruptions to existing workflows.
See also: How to Select a Streaming Platform: A Practical Guide
Enabling HTAP for Dynamic Business Outcomes
The integration of lakehouse formats into streaming infrastructure is a key enabler of true HTAP capabilities. By breaking down the barriers between operational and analytical data processing, organizations can achieve more dynamic and immediate business outcomes.
Real-time data from streaming sources can be instantly combined with historical data in the lakehouse, providing a comprehensive view of business operations. This enables more informed decision-making and faster response times to changing market conditions. For example, a financial services company could use this approach to detect fraudulent transactions in real time while simultaneously analyzing long-term trends to improve their fraud detection models. The ability to seamlessly blend real-time and historical analysis creates new possibilities for innovation and competitive advantage.
What’s Next for Data Streaming Infrastructure?
As data continues to play an increasingly central role in business operations and decision-making, the importance of efficient, flexible, and scalable data architectures will only grow. The integration of lakehouse formats with streaming infrastructure represents a significant step forward in meeting these evolving needs.
Organizations that embrace this unified approach to data management will be better positioned to derive value from their data assets, respond quickly to changing market conditions, and drive innovation through advanced analytics and AI applications. While the adoption of these technologies may require some initial investment and organizational changes, the long-term benefits in terms of improved data accessibility, reduced costs, and enhanced analytical capabilities make it a compelling proposition for forward-thinking businesses.