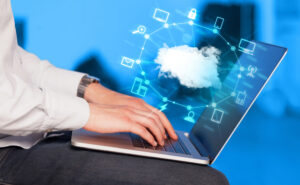
The ability for companies to benefit from the convergence of cloud computing and generative AI will hinge upon putting both technologies to work on their data.
In just a few years, cloud computing has steadily grown from industry disruptor to industry staple. The ability for companies to access the necessary IT and technology configurations for operation via a more efficient cloud service model instead of expensive on-prem builds has become nearly unavoidable. In fact, recent research from Gartner predicts that by 2028, cloud computing will transition to ‘business necessity’ status, the highest and final stage for emerging technology, and the moment it becomes a necessary component for maintaining business competitiveness.
The demands for physical data storage and its retrieval have transitioned to the cloud because of its centralized and efficient data storage model and the integrity, security, and accessibility it provides for a company’s data, known as cloud data. Due to the growing variety of providers in cloud computing, and organizational nuances with regards to data governance requirements, the specifics of cloud data management can vary greatly from organization to organization.
Yet, despite this variability, the success of every organization’s cloud data management strategy will ultimately converge on a single point: the data itself.
See also: Where to Train Your AI Dragon
Focus on the data
No matter how well-configured, even the most elegant cloud data management solution will prove to be a gigantic waste of time and resources if the data is not well-prepared or useful to the business. Just as cloud computing’s potential is only as good as its underlying tech stack, cloud data management is only as good as its underlying data.
I would argue that fine-tuning the tech stack in order to create an environment where cloud data management workflows are not just operational but fully optimized can become even more critical than the tech stack itself.
Data (e)quality
Not all data are created equal. Furthermore, not all data is ready for prime time. Data must be cleaned, structured, and prepared so it is ready for analysis, the process of generating the much sought-after data-driven insights. Only data that has been properly cleaned and prepared should be used for analysis, while poor quality and dirty data must be avoided at all costs. Bad data can only lead to bad decisions. Dirty data costs the U.S. $3 trillion per year, according to Harvard Business Review’s estimate.
The importance of data preparation
Data preparation is critical for successful cloud data management, enabling companies to automate all stages of data analytics – from data extraction and preparation to analysis and reporting and even applying generative AI.
Ultimately, many organizations still rely on outdated manual methods for data preparation. These processes are a tedious and error-prone slog that creates bottlenecks in data availability — an especially cruel fate for cloud data and its reputation for quick ease of use. Such challenges can single-handedly hamstring the innovative potential that was intended by a forward-looking cloud data management strategy.
See also: Break Down Silos with Seamless Cross-Cloud Data Sharing
Generative AI rolls up its sleeves
The good news is that generative AI has capabilities that can supercharge data preparation and streamline the entire data analytics lifecycle. While companies have already been applying machine learning to data preparation, there is now a new opportunity for even further efficiency gains.
Generative AI makes analytics tools easier to use, including those used for data preparation. Much of this is driven by the natural language interfaces that make using analytics processes much easier, as users can execute complicated analytics tasks using basic English instead of having to learn Python or SQL, for example.
Generative AI also substantially improves automation quality for analytics and can be applied across the entire data analytics lifecycle – from the extract, load, and transform (ELT) through the data preparation stage. When applied, generative AI:
- Streamlines the ELT process: Predictive algorithms and LLMs can be applied to optimize data extraction, intelligently organize data during loading, and transform data with automated schema recognition and normalization techniques.
- Accelerates data preparation through enrichment and data quality: AI algorithms and LLMs can predict and fill in missing values and identify and integrate external data sources to enrich the dataset, while advanced pattern recognition and anomaly detection ensure data accuracy and consistency.
Is cloud computing ready for AI?
The mad dash to onboard generative AI has kicked off an arms race to build out the physical infrastructure to support it. The flipside of cloud computing’s popularity over the past decade is that it puts cloud service providers squarely ‘on the hook’ for building out their clouds to handle the compute required by its clientele. As companies increase investment in generative AI, cloud providers will continue to rush to meet demand. While an undertaking of this magnitude is not without its challenges, cloud providers are increasingly confident they can rise to the challenge and provide enterprises with what they need to capture the AI boom.
The ability for companies to benefit from the convergence of cloud computing and generative AI will hinge upon putting both technologies to work on their data. With cloud providers poised to provide the physical and technical requirements to bring cloud computing into the generative AI era, organizations must rise to the occasion and prioritize cloud data management practices: the success of both cloud and AI initiatives depends on it.