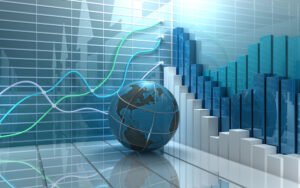
A talk with KX CEO Ashok Reddy about the need for a single platform that includes processing, analyzing, building models, and visualizing to enable low-latency decisions in capital markets organizations.
Capital markets organizations face many data and analytics challenges in making faster trading and financial transaction decisions. Those striving to improve operations and make use of the ever-growing availability of historic and real-time data need a multitude of technologies to make sense of the data and develop predictive models.
RTInsights recently sat down with Ashok Reddy, CEO of KX, to talk about what’s going on in the market, the complexity of traditional approaches, and the need for an approach that includes processing, analyzing, building models, and visualizing all in one single platform.
Here is a lightly edited summary of our conversation.
RTinsights: What goes into making fast but confident decisions?
Ashok Reddy: The first thing is ensuring a data-driven approach with high-quality data. When we talk about AI, it’s really about lowering the cost of cognition — helping to make decisions faster and more accurately. For AI to effectively assist in decision-making, the data must be AI-ready — meaning it’s relevant, clean, trusted, and representative of the context in which it will be used. In capital markets, this might include pre-processed historical price data, market sentiment from social media, or economic indicators that have been normalized for easy integration into predictive models.
Making decisions in capital markets is fundamentally about predicting the future. This isn’t just about analyzing what’s happening now but using data to forecast what will happen. The decisions that are hardest to make are those that involve future outcomes, and that’s why we look at decision-making as a forward-looking activity. The data you use today should help you create models that are not just accurate but can evolve as new information comes in. This is where AI becomes crucial — it helps refine these models continuously, improving their predictive capabilities.
In practical terms, you must factor in time. What can we learn from historical data to make better decisions today? And as new data comes in, how do we incorporate that into our existing models? This is why decisions are, at the end of the day, about creating and refining models that represent our understanding of the future. These decision models need to be accurate, learn from past events, and adjust as new data becomes available, ensuring that predictions remain precise and reliable.
RTinsights: What technologies do you see capital markets adopting? Why should they prioritize these technologies, and how can they be best applied?
Ashok Reddy: Capital markets thrive on information and the edge it provides. To maintain this edge, organizations need technologies capable of processing diverse types of data — market data, time series data, and more. The data should be processed regardless of its form, whether it’s structured or unstructured. Speed is crucial; the technology must handle high-frequency, real-time data while integrating large volumes of historical data.
When it comes to technology adoption, capital markets are driven by the need for speed and accuracy. Companies are looking for technologies that can handle vast amounts of data in real-time. The ability to process different types of data in a seamless manner is critical. For example, when data comes in from market exchanges, you need technologies that can handle not only the speed but also the volume and variety of data. This includes structured data, like market prices, as well as unstructured data, like news feeds or social media posts.
Another key aspect is backtesting, which is essential for validating trading strategies. Before deploying a strategy in the market, you want to simulate how it would perform under different scenarios. This requires technologies that can handle historical data in a way that’s both comprehensive and efficient. The ability to backtest with data from multiple sources — whether it’s historical market data or alternative datasets like video streams or news articles — can provide that crucial edge.
The regulatory environment in capital markets is strict, so the technologies they adopt need to be auditable and explainable. This isn’t just about making sure the models work but also about being able to explain how they work to regulators. Companies need to ensure that the technologies they use are compliant with regulations and that they can provide clear, auditable explanations of their predictions and decisions.
RTinsights: What challenges do such companies have adopting and integrating these technologies into their operations and workflows?
Ashok Reddy: One of the biggest challenges is the sheer amount of data capital market companies have to handle — not just the volume but also the speed and variety of the data. They need to manage both structured and unstructured data at high speeds. The second major challenge is the lack of out-of-the-box solutions that can handle everything from data processing and analysis to storage, reporting, and regulatory compliance. Even if they start with open-source solutions or different tech stacks, there’s no full-stack solution available.
The reality is that many companies end up having to build their own solutions, which can be incredibly costly and time-consuming. Take, for instance, large financial institutions like JP Morgan Chase, Bank of America, and Morgan Stanley — they spend billions of dollars on technology every year because they need to build these solutions from the ground up. And even with these resources, they face challenges like integrating multiple technologies, ensuring high performance, and meeting regulatory requirements.
A significant challenge is that there are no turnkey solutions that address all these needs simultaneously. Companies might start with a high-performance solution for processing large volumes of fast data, but then they find that the data ingestion process is too slow or the storage system can’t handle the volume. This leads to a mismatch of technologies and inefficiencies across the board.
Then, there’s the issue of data movement. Traditional technologies often require moving data from one place to another for processing or analysis, but with the massive volumes of data involved in capital markets, this becomes impractical. Companies need solutions that allow them to bring the analysis to where the data is stored rather than the other way around. This reduces latency and allows for real-time processing, which is critical in a market environment where timing is everything.
RTinsights: How does KX help?
Ashok Reddy: KX was built from the ground up to handle the unique demands of capital markets, particularly the need for processing high-frequency, vast volumes of data in real-time. Specifically, KX processes and analyzes data in memory, with data stored in a columnar format to ensure speed and efficiency. This approach ensures that data quality is maintained throughout the process.
KX integrates processing, analyzing, and storing data in one place, which simplifies operations and enhances performance. You can take existing data and play it back to test strategies, using decades of historical data or even just last year’s data. It’s like having a time machine — you can go back and replay scenarios or fast-forward to see potential future outcomes. This capability is crucial for developing and validating trading strategies before they’re deployed in the market.
We also focus on maintaining the highest data resolution even during compression, avoiding the common pitfalls where compression leads to a loss in accuracy. KX provides a unified platform for building, testing, and deploying models. This isn’t a separate data science platform; it’s integrated within the same system, allowing quant research teams to perform ad-hoc and dynamic queries. This flexibility means you can explore different problem spaces without being limited by the initial design of the database.
KX is built to be both auditable and explainable, which is critical in the highly regulated environment of capital markets. Our platform is designed to comply with stringent regulations such as MiFID II and Dodd-Frank, ensuring both high performance and the necessary auditability and transparency that regulators demand. This enables companies to rapidly develop and deploy their strategies while also demonstrating to regulators how these strategies operate and how they align with relevant regulations. As regulatory scrutiny intensifies across the industry, our platform provides the tools needed to meet compliance requirements.
RTinsights: Could you give some examples, use cases, etc?
Ashok Reddy: The first use case is developing alpha — an algorithm or model that beats the market. This typically involves quant research, backtesting, and bringing in new data to power testing. It’s a front-office use case directly tied to the company’s P&L, focused on trade ideation, research, trade execution, and risk management.
For example, a quant research team might develop a new trading strategy that they believe can outperform the market. Using KX, they can backtest this strategy against past data to see how it would have performed in various market conditions. They can also fast-forward using real-time data to predict how the strategy might perform in the future. This kind of comprehensive testing is crucial for developing strategies that can consistently generate alpha.
For the middle office, use cases include fraud detection and trade surveillance, ensuring that while pursuing profit, risk is effectively managed. Fraud detection, for instance, involves analyzing large volumes of data in real time to identify suspicious patterns or activities. With KX, companies can monitor transactions as they happen, flagging any anomalies that might indicate fraudulent behavior. This allows them to act quickly to prevent fraud before it impacts the business.
Trade surveillance is another critical area where KX can be applied. In a highly regulated environment, companies need to ensure that all trades are compliant with regulatory requirements. KX enables real-time monitoring of trades, ensuring that any potential issues are flagged immediately and dealt with before they escalate.
The back office focuses on efficiency and price-to-performance, ensuring that technology investments provide a solid return. Standardization across shared services helps drive P&L while keeping costs under control. For instance, KX’s ability to compress large volumes of data without losing resolution or accuracy means that companies can store more data in less space, reducing storage costs. At the same time, KX’s high-performance processing capabilities mean that companies can do more with less, reducing the overall cost of ownership while still delivering the performance needed to stay competitive in the market.
Additional Resources
AI-driven Real-time Decisions in Capital Markets (Webinar)
KDB.AI – Enabling AI-driven Data Immediacy for GenAI Applications (Analyst Report)
Capital Market Use Cases That Require AI, Digital Twins, and Data Immediacy