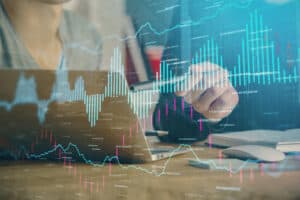
AI serves as a valuable ally to businesses, providing multiple advantages in the growing need for informed data analysis.
Businesses face the grueling task of analyzing large amounts of dispersed and siloed data on a daily basis. With time pressure and tight budgets, organizations struggle to innovate and keep pace with the evolving landscape of data. The need for modern technologies, including artificial intelligence (AI), to streamline these operations is becoming increasingly important. Let’s explore the unique ways AI technology is helping to address complex data analytics challenges that business leaders are facing – helping them enhance operational efficiency and ushering in a new era in data analysis.
Addressing Key Data Analytics Obstacles in the Business Landscape
Business leaders face a variety of challenges that affect the widespread distribution of accurate, timely insights, ultimately decreasing operational efficiency. Businesses across a range of industries have identified common issues such as limited time and budget, developing effective data-centric environments, and fewer siloed data sets.
Organizations face difficulties with data automation, governance, and integration, which impact their ability to execute data operations on fixed budgets. In an attempt to solve these issues, enterprises often engage in extensive data infrastructure projects, which result in higher costs and longer timelines. In contrast, a more balanced approach companies can adopt includes the combination of common solutions on the market for efficiency and reducing expensive custom development for projects.
One of the most prominent hurdles commonly discussed among industry leaders is the development of data literacy and the overall fostering of a data-centric environment across an organization. Data management can be complex and intimidating to business leaders, making it challenging for them to break the habit of making uninformed decisions instead of using insights. Nevertheless, the responsibility to tackle this challenge remains with business leaders, who can take proactive measures by engaging with technology teams to instigate transformation and ensure that user-friendly, intuitive interfaces are in place for business users. In particular, organizations can harness the power of AI to enable easier access to data, deliver personalized insights, and provide user-friendly analytics tools.
Organizations often face the irony of encountering siloed and dispersed data sets, which creates the perception of a scarcity of data. However, upon further analysis, they often find a plethora of usable information. Even as organizations continue to uncover information, they face the additional challenge of ensuring that this ‘data liberation’ doesn’t unintentionally introduce new hurdles, such as data warehouses necessitating specialized teams for access and ongoing support. To enhance data interoperability, organizations can now deploy modern technologies like AI to automate data integration and analysis, helping them bridge data silos, identify correlations and unveil valuable insights without requiring specialized teams to perform extensive or manual data management procedures.
See also: Gartner Predictions: My How Far Data Analytics Has Come
AI/ML’s Progressing Role in Data Analytics
Enterprises have integrated AI into data analytics software for quite some time, with a particular focus on two key AI forms: machine learning (ML), predominantly deployed for forecasting, and natural language processing (NLP), heavily leveraged for generating insights.
However, in recent years, businesses have seen significant AI and ML advancements, with innovations such as Chat-GPT paving the way for a new standard of technology. Now, these technologies are helping organizations uniquely solve specialized issues tailored to their business needs.
Using AI to Solve Unique Business Needs
When it comes to addressing specific data management challenges, AI particularly excels in the curation of mock data – enhancing the ways in which businesses deliver data. Using AI to generate mock data offers a significant advantage to businesses: it enables them to test and demo data products without the need to collect real data from users, making it an invaluable tool for those developing new data products or testing new features for existing data products. This sort of data generation, which includes generating data like fake reviews, helps create a fast and effective data product, providing a valuable solution to help organizations develop data literacy and make more insight-informed business decisions.
As an illustration, my coworkers and I used AI to fabricate counterfeit evaluations for an imaginary Antarctic amusement park, requesting the algorithm to inject a bit of wit. The algorithm’s outcomes encompassed statements like: “Avoid the cafe at Antarctica Aquarium. First, it’s weird to serve fish and chips at an aquarium; don’t you know the fish are watching? Second, how is it possible I can’t get ice in my Coke? One star.” Additionally, the AI-generated counterfeit transactions for the park’s gift shop, including items such as the “Mate for Life: Marriage Advice from Emperor Penguins, $45.00.”
Guess, Grind, or AI?
As corporations leverage data to improve business forecasting, there is a concept my colleagues and I often use that aligns well with the methods that can be deployed in this process: ‘Guess, Grind, or AI.’ Organizations can either opt to make educated guesses, trust their instincts, or delve into a comprehensive spreadsheet analysis to confront difficult scenarios. These scenarios may involve factors such as historical school holiday data, weather changes, unforeseen events, growth rates, and the impact of events like the pandemic. Alternatively, they can use ML.
When forecasting, businesses can leverage ML to deliver more granular, highly automated, and precise predictions, as opposed to using manual projections. ML solves the inherent knowledge risk of organizations operating on “gut feel” and “experience” to make predictions. ML can also pick up on the subtleties and nuances invisible to the human eye, such as multiple factors playing out in parallel.
As an example, a large visitor attraction, such as a zoo, can use ML to predict visitation rates by factoring in real-time and anticipated impacting events. These include subtleties such as the time of year, day of the week, competing local events, and even weather conditions. In addition, ML can be employed to assess the impact of each factor and calculate the discrepancies between forecasted and actual visitation for attribution purposes.
The Future of Data Analytics and Analysis
While AI can aid in enhancing and optimizing data analysis, it doesn’t supplant the indispensable traditional role of human expertise and software engineers. Exceptional technology necessitates leadership and creativity and adept navigation of intricate ecosystems and stakeholders—an innately human skill.
In the end, however, AI serves as a valuable ally to businesses, providing multiple advantages in the growing need for informed data analysis. By harnessing AI, organizations can expedite the process of making accurate, well-informed decisions. AI-driven ML substantially elevates forecasting precision, unlocking deeper insights into intricate scenarios and mitigating knowledge-related risks.