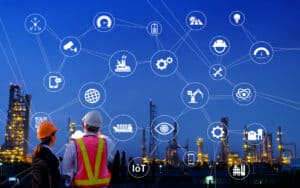
By analyzing real-time data generated by IoT devices, organizations can gain actionable insights to drive informed decision-making, enhance operational efficiencies, and create new revenue streams.
The Internet of Things (IoT) has revolutionized how we interact with the world around us, and the shift to connected devices continues to accelerate. In fact, by 2030, 75% of all devices are projected to be IoT. While the sheer volume of data generated by these devices is staggering, storing, and sharing this data for real-time monitoring and analytics presents a significant challenge. Organizations that can harness the power of this data will be able to identify meaningful insights that lead to more informed decisions and increased efficiencies.
Although real-time data sharing with third-party analytical tools and other systems is often crucial to developing insights, doing so raises challenges around network latency, bandwidth limitations, and the need to centralize information from multiple locations. Sharing data can pose security challenges, so organizations must take proactive measures to safeguard their data and networks from potential cyber threats.
Despite the challenges, the continued growth of IoT devices shows that the benefits outweigh the drawbacks. Organizations must overcome these challenges and invest in secure, seamless real-time data-sharing solutions. In doing so, they can unlock the full potential of IoT and gain a competitive edge in their respective industries.
But what do companies need to know to see significant benefits?
See also: 5G IoT Connections To Surpass 100 Million By 2026
Technical Challenges
Network latency presents a significant obstacle to real-time data sharing in IoT, particularly for applications requiring instantaneous real-time monitoring or analysis. This challenge becomes more pronounced in low bandwidth connectivity scenarios, where legacy sensors not equipped to manage modern IoT data need help handling large, critical data volumes. To address these challenges, organizations can employ data replication strategies.
One approach is to utilize data replication tools capable of storing data locally during periods of poor reception. This ensures that critical data is not lost and that it can be automatically stored, transmitted, and processed when network bandwidth becomes available. In scenarios where devices are outdoors or located apart from office buildings and lack internet infrastructure, relying on a low bandwidth network for reporting data, such as cell towers, is the norm. Additionally, processing data at the point of collection with modern data replication tools enables on-site data processing, allowing for transmitting a smaller batch of highly relevant data, such as aggregated or anomalous data, vs. all of the raw collected data.
Finally, relational databases, commonly used for data management, may not be optimal for data replication and real-time analysis. Purpose-built time series databases offer superior capabilities in managing streaming data, making them ideal for real-time monitoring and analysis. These databases treat data as a continuous stream, ready to be moved or processed. Organizations can access unlimited storage and processing resources by pairing a purpose-built time series database with a cloud-native approach, enabling seamless scalability and efficient management of network latency challenges.
See also: How to Combine Tech Debt And Modernize Legacy Software
Centralization Challenge
Centralization is another significant concern for IoT data, especially for large manufacturing companies or utilities that have been in business for years. These organizations often built or acquired sites over time, with separate teams implementing data systems with different technologies and processes. Exporting data from each site often requires several semi-manual steps incompatible with real-time analytics. Once the data is collected organization-wide, a significant amount of clean-up is required to align the data and get it into a format that can be shared with other tools or systems.
Fortunately, new technology and approaches allow organizations to address these challenges without a complete rip-and-replace of their existing infrastructure. Even within an infrastructure that’s generally viewed as a “closed” system, new APIs and data connectors can work with legacy data historians to centralize data from multiple sites and automatically address the data alignment issues that inevitably arise. Once the data is cleaned up, data subscription services give system admins finely-tuned control over who can access the data, including limitations to specific database segments. By adopting these tools, companies can centralize their data-sharing systems and ensure their devices work together efficiently.
See also: Game of Protocols: How To Pick a Network Protocol for Your IoT Project – Part 2
Security Concerns
The explosion of data generated by IoT devices has caused significant global concerns about security due to the ease of accessing and sharing data with multiple parties. Specifically, data privacy, as connected devices create numerous entry points that can leave sensitive information vulnerable to hacking.
It has even become a national priority. Recently, the White House has taken a more aggressive stance to prioritize securing IoT and outlined plans to mature its security measures in the latest National Cybersecurity Strategy. These actions aim to strengthen cybersecurity measures and safeguard data privacy for IoT devices. Organizations must proactively invest in robust device security measures and adhere to the newly introduced guidelines to safeguard and authorize their data effectively.
Although on-premises systems were historically viewed as the gold standard for security, there’s debate as to whether they’ve been surpassed by Amazon, Google, and Microsoft cloud offerings. These giant tech companies have a deep bench of security experts to work with and invest significant resources into safeguarding their customers’ data. By adopting robust security measures and exploring secure cloud offerings, companies can establish a strong foundation for protecting their IoT devices and mitigating security risks.
Interoperability Challenge
Interoperability is also essential for the seamless operation of IoT devices and systems. With the growing number of IoT devices, there is a pressing need for standardized communication protocols that can facilitate interoperability between devices and systems. Lack of standardization can hinder data sharing between devices and systems, which can impede the potential of IoT. Organizations can leverage open standards such as MQTT and CoAP to overcome this challenge to enable cross-platform communication between devices and systems.
The sheer volume and velocity of data generated by IoT devices can be overwhelming, making it hard for organizations to extract meaningful insights. However, integrating advanced analytics powered by machine learning can help organizations make sense of the data and derive valuable insights. By analyzing real-time data generated by IoT devices, organizations can gain actionable insights to drive informed decision-making, enhance operational efficiencies, and create new revenue streams.
Real-time data sharing in IoT holds great potential for organizations to drive meaningful insights and gain a competitive advantage. However, it also comes with significant bandwidth, centralization, and security challenges that must be addressed. By investing in new IoT connectors, implementing standardized communication protocols, adopting advanced analytics tools, and prioritizing security and compliance, organizations can overcome these challenges and unlock the full potential of IoT.