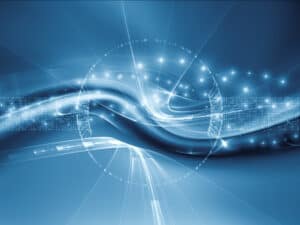
With the potential to democratize AI and ML, AutoML is the answer many enterprises across industry verticals have been seeking to take AI projects from pilots to scaled deployments.
Adopting Artificial Intelligence (AI) is no longer just to gain competitive advantage; it has become table stakes for mere business survival. However, today’s acute shortage of data scientists combined with the continuous effort to automate laborious tasks is posing unprecedented challenges for enterprises. Automated machine learning (AutoML) is poised to help.
Why? Traditional machine learning (ML) is a time-consuming, arduous, and iterative task that involves data cleansing and preparation, algorithm training, validation, etc., to imitate the way that humans learn to make predictions or decisions without being explicitly programmed to do so. The sheer effort involved is resulting in dampening the adoption of AI at enterprises to some extent begging the need for a continuous effort to automate these tasks by building more intelligent ML procedures and algorithms.
Automated machine learning provides the methods and processes for automating virtually every stage of the ML process, from processing raw datasets through to building machine learning models that are ready for deployment. It goes beyond simple automation of tasks by accelerating the entire process of building ML and deep learning models. AutoML automates several aspects of the ML processes, including the identification of the best-performing algorithm from the available features, algorithms, and hyperparameters.
See also: 3 Considerations for Adding Real-Time ML to Applications
The advantages of AutoML
The most obvious benefit of AutoML is that by eliminating mundane tasks such as data cleansing and preparation, AutoML frees up key technical human resources to handle projects that require human intervention, such as value-adding analysis and in-depth evaluation of the best-performing models, etc. It also allows technical experts such as data scientists to remain focused on business-critical tasks.
The complexity of today’s ML models has virtually turned them into black boxes that provide very little visibility into what takes place inside and what is impacting the results. By taking the human element out of some of the more iterative ML processes, automating ML processes enables businesses to focus on eliminating data biases, reducing human errors, inspiring trust by providing transparency on how the model functions, and improving overall model efficiency.
Year-over-year, the value of data continues to increase. However, to make sense of the ever-increasing amount of data and use it to gain insights and make decisions, the demand for data scientists continues to escalate. In fact, a report by the UK’s Royal Society issued a warning that the demand for data scientists and data engineers had more than tripled over the last five years, leaving businesses without the professionals needed to unlock the potential of ML and AI. With data scientists becoming a rare commodity, AutoML is allowing business users to develop and execute their own data models with minimal training in data science, ML, or AI.
This democratization of AI bridges the key skills gap, enabling those familiar with analysis tools like simple Excel and an interest in data analysis to become citizen data scientists. Through AutoML, citizen data scientists can easily automate tasks like data pre-processing, feature engineering, and hyper-parameter turning. In addition, instead of relying on the traditional rebuilding of the different models driving AI, AutoML allows the reuse of features.
Aside from democratizing ML, AutoML accelerates the speed of training multiple models while improving accuracy. It makes ML more scalable by enabling multiple ML models to be trained simultaneously, optimizing the overall ML processes. Additionally, by introducing an AutoML platform that doesn’t require code at the AI platform, enterprises can reap benefits for many of their team members, such as business leaders, business analysts, software engineers, and data scientists.
- Business leaders can accelerate AI adoption, drive innovation with AI-driven decision analytics, scale up or down depending on the business’ goals and usage, reduce technology investments and infrastructure management costs, and improve data science team collaboration.
- Business analysts will be able to enhance productivity, efficiency, and return on investment (ROI) with AI that doesn’t require coding, and gain faster data insights while minimizing dependence on the IT team, and quickly build ML pipelines.
- Software engineers will benefit from being able to expedite experiments, create faster ML pipelines, gain instant insights with intuitive visualizations, and build robust and transparent AI models more rapidly.
- From a data scientist’s perspective, they’ll have the ability to create complex models and easily run multiple AI experiments, gain instant insights, and quickly build robust and transparent AI models.
By making data science accessible to all users, it enhances the collaboration between domain and technical experts, which encourages teams to remain focused on business value and not get lost in the technical maze of the implementation. Over time this will help enterprises remove silos and promote collaboration across users.
Take AI projects from pilots to scaled deployments
For many enterprises, AI projects remain in the pilot stage longer than they should or need. This is primarily due to the lack of data scientists, slow progress in ML processes, and even the lack of coordination between the business and data teams. However, this is changing. A Gartner study determined that 75 percent of organizations will shift from piloting to operationalizing AI by the end of 2024, driving a 5x increase in streaming data and analytics infrastructures. Additionally, 50 percent of enterprises will devise AI orchestration platforms to operationalize AI. However, these predictions rely on AutoML technologies. Without it, AI projects will likely to remain much longer in the limbo pilot stage.
With the potential to democratize AI and ML, AutoML is the answer many enterprises across industry verticals have been seeking to take AI projects from mere pilots to scaled deployments. The availability of AutoML platforms plays a central role by increasing the efficiency of data scientists by allowing them to focus on higher-value tasks. Since these platforms can automate every step of the data science lifecycle, including feature engineering, algorithm selection, and hyperparameter tuning, enterprises can enhance operational efficiency and improve time to market.
What does this mean for companies that don’t have the professional skillsets required by ML and AI? With AutoML democratizing AI, a greater number of companies will be able to reap the benefits of AI with lower investments. However, it needs to be noted that the democratization of these technologies doesn’t instantly turn every employee into a citizen data scientist and definitely not into a data scientist. To get the most from AutoML, businesses need to provide their potential citizen data scientists with training on how to interpret and analyze the data, as well as provide instructions on data governance to ensure that the data is being reliably used. AutoML platforms and tools should become a key component of any enterprise, big or small, interested in seeking ROI on AI/ML solutions and moving them from the pilot stage to the scaling stage.