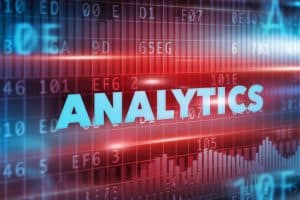
The concept of an end-to-end data analytics chain is already proving itself in business settings, with leaders reaping substantial rewards.
Despite the hype with rosy vendor and analyst pronouncements, the state of data and analytics is still dismal – hampered by latency and data silos. It’s time to look at a new way of organizing analytics environments.
That’s the word from analysis published by Business Application Research Center (BARC), based on ongoing surveys of BI-consuming enterprises from across the globe. “Too seldom do companies generate relevant insights as quickly as they desire and need to,” the report’s authors state. “Analytics content must be created in an iterative manner and it must not be impeded by the restrictions caused by data silos.”
While there has been endless excitement about high-level analytics delivered through artificial intelligence, it’s time to pay more attention to the front end of the process. That is, the business intelligence (BI) tools that bring all the insights together for digestion by business decision makers.
“When BI started being industrialized in the 90s, it was largely considered an IT topic as it required fundamental technical expertise,” according to the BARC authors. Business users got around IT with shadow BI, initially powered almost exclusively by Excel. “Change came a decade later with the advent of user-friendly self-service BI and visual analytics. Unfortunately, a general lack of governing capabilities, originally perceived as guarantors of flexibility, and the dissemination of analytics into all corners of modern companies overstrained the approach.”
See also: Putting More Intelligence into Business Intelligence
To overcome these issues, the BARC authors urge adoption of “vertically integrated data and analytics software,” which “couples the flexibility required for quick insights with governance features for scaling decentralized self-service and blending it with central delivery.”
Such vertical integration – extending from the backend database to the front-end analysis tool – provides companies the capability to “convert data into insights effectively throughout the organization, enabling them to support decision-making and drive innovation,” the analysts note. Tools include integrated data and analytics software as well as reporting and data preparation solutions. The good news is these end-to-end tools “are quicker to implement through the cloud and easier to use than ever thanks to machine learning-based augmented guidance features.”
The concept of an end-to-end data analytics chain is already proving itself in business settings, the BARC team says. “While laggards have not fully bought into the benefits of the concept yet, leaders have already acted and are reaping substantial rewards.”
This is a clear shift from the paradigm promoting efficiency through horizontal integration, which consisted of “creating all reports in a single tool and storing all data in a common data warehouse was meant to boost efficiency,” the report notes. “Horizontal integration curbed the innovative capacity of companies to use their data.”
Advanced and predictive analytics, machine learning and AutoML all are more effectively supported in an end-to-end data supply chain.
Moving to an end-to-end data supply chain requires rethinking the way data is organized and delivered across the enterprise. The BARC analysts make the following recommendations to move in this direction:
- Identify requirements that can be covered within an end-to-end platform. “It must not generate additional isolated data silos that limit the innovative power of analytics.”
- Reassign developers to train, coach and advise business users to master their own analytics challenges. “While business analysts and data scientists move to center stage in decentralized analytics, the contribution of developers and data engineers to smooth operations cannot be overestimated.”
- Include data preparation facilities in AI efforts. “Consider requirements of automating decisions even though they may not be crystal clear yet. Operationalizing, deploying and monitoring analytics and ML models in production will be relevant for all companies sooner or later and many are not prepared properly yet.”
- Catalog all the analytics assets created. “A comprehensive overview of reports, dashboards, data sets and analytics models is the oil to get the engine of your analytics processes running smoothly.”
- Appreciate the potential of integrated software with exhaustive metadata collection. “These tools deliver common and extensible semantic models, collect compelling usage statistics and provide transparency into where data is sourced from, how it is transformed and where it is presented.”
- Consider cloud-based analytics solutions. “Software as a service combines the advantages of practically unlimited scalability, quick set up and resource-efficient operation. For modern analytics, the cloud is a platform that can deliver.”