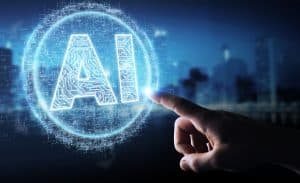
Zoldi makes the case that Responsible AI can address concerns about model accuracy, ensure appropriate uses, remove doubt about assumptions, and overall elevate AI use in business today.
Explainable AI is a favorite topic of Scott Zoldi, Chief Analytics Officer at FICO. It has come up in several conversations we’ve had with him, in a previous article he penned for RTInsights, at last year’s FICO World event, and in his keynote address at the recent FICO Virtual Event webinar.
However, Explainable AI is part of the much broader need for Responsible AI, which covers a range of topics data scientists must address to ensure their models are accurate, reflect real-world conditions, and accepted by their organizations.
He cites what he calls data scientist cowboys as one example of why companies need to focus on Responsible and Explainable AI.
Data scientist cowboys have appropriate training but fail to understand the correct way to handle machine learning and artificial intelligence. Typically, cowboys are delighted by the results produced by their software of choice, xgboost, which they wield like a hammer while approaching every data science problem as if it’s a nail.
Zoldi noted how xgboost is a risky hammer to wield – it relies on gradient boosted decision trees (GBDT), in which the first tree to fit a given dataset produces a second tree, which produces a third, and so on. The results appear to guide data science cowboys to their goal but are produced by a distracted algorithm that is over-trained at best and risks crashing the user’s system at worst.
Enter Responsible AI
In his keynote speech, Zoldi provided an overview of the different aspects of Responsible AI and what goes into each. In particular, he shared the following.
Explainable AI is a key issue that many businesses are working on. They rely on AI model predictions to take actions. However, they may not fully understand the underlying models and (for lack of a better word) limitations of those models.
The issue of explainable AI is getting extra attention these days due to major business disruptions brought on by the COVID-19 pandemic. Many predictive models are presented and used as simple black boxes. If a model was developed using a certain dataset and data changes, what would the implications be on the prediction? Also, what if the wrong machine learning models are used to make the predictions?
Take a model that tries to predict customer payment waiver requests. The normal distribution for those requesting one, two, or three waivers fit certain distribution patterns. Do these patterns hold up under current economic conditions that have seen more than 40 million people filing unemployment claims in the U.S., global stock markets having suffered dramatic falls, and the Dow Jones reporting its largest-ever single day fall of almost 3,000 points on March 16, 2020?
Without detailed knowledge of the model’s workings (what data and which algorithms were used?), there is no way to convey a level of confidence in the predictions.
Simply put, Explainable AI is a field in machine learning that tries to address how black box decisions of AI systems are made.
Efficient AI focuses on what Zoldi says is “preparing for the journey.” What that means is data scientists must consider lifecycle issues of developing the model, ensuring data is accurate and relevant throughout the model’s lifetime and updating the model when needed.
Some ways data scientists can address efficient AI issues are to:
- Use standard machine learning development pipelines
- Use open-source machine learning libraries to “clean, wrangle, and munch” data
- Where possible use tested algorithms, with the suggestion of licensing proven models, verses building them from scratch
- Reuse existing models that are battle-proven
Robust AI: Not to be confused with the company with the same name, robust AI addresses weaknesses and deficiencies that might make a model less useful, inaccurate, or degrade over time.
Factors that help ensure and contributed to robust AI are:
- Proper problem formulation
- Encompassing historical data
- Sufficient volume of quality tags
- Solid performance definition
- Proper model selection
- Train / test / hold out data
- Model scenario / stability testing
- Model governance
Ethical AI seeks to ensure models do not intentionally or unintentionally exhibit biases. A big concern about artificial intelligence is potential bias by algorithms that reflect the limited worldviews of programmers. Bias can also enter through datasets that skew results.
In late 2019, Apple faced allegations that the Apple Card used an algorithm that discriminated against women in credit-scoring evaluation. The issue was raised after Apple’s co-founder Steve Wozniak and entrepreneur David Heinemeier Hansson received credit limits 10 to 20 times higher than their wives.
Ethical AI is getting lots of attention at the corporate level. A State of AI in the Enterprise survey from Deloitte found that 32% of executives ranked ethical issues as a top-three risk of AI.
Bottom line
Data science cowboys who do not take care when implementing AI or companies that ignore issues related to a model’s biases, use of suitable algorithms, and model degradation over time can cause problems. AI models will return erroneous results, companies will lose confidence in predictions, and poor business decisions will be made.
Zoldi makes the case that Responsible AI can address concerns about model accuracy, ensure appropriate uses, remove doubt about assumptions, and overall elevate AI use in business today.