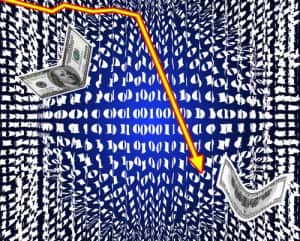
Data quality and labeling issues devour budgets and schedules, new study says.
Nearly eight in 10 organizations rolling out AI and machine learning experience project delays, according to a new global survey by Alegion and Dimensional Research. A whopping 96% of respondents blamed problems with data quality, model confidence and data labeling needed to train AI.
These data issues have caused enterprises to blow through their AI project budgets and deadlines.
That’s obviously unwanted news for the 84% of enterprises that believe investing in AI will lead to greater competitive advantages (Statista). IDC predicts worldwide spending on artificial intelligence (AI) systems will grow 44% in 2019, reaching $35.8 billion.
“While enterprises see AI as critical to differentiating themselves within the market, they encounter challenges early in the process, primarily around accurately and efficiently labeling and annotating enough data to train their algorithms,” says Nathaniel Gates, CEO and co-founder of Alegion. The Austin, TX-based company provides training data for ML projects.
To get AI systems off the ground, training data must be voluminous and accurately labeled and annotated. With AI becoming a growing enterprise priority, data science teams are under tremendous pressure to deliver projects but frequently challenged to produce training data at the required scale and quality.
See also: How to Merge Machine Learning and Data Prep
Gates predicted that as enterprise data science teams gain more experience with machine learning projects, they’ll be more likely to offload such activities as data labeling, and model validation and scoring as way to speed their path to model deployment.
Snapshot of Global AI/ML Projects
The survey included more than 200 business stakeholders and data scientists involved in active AI and ML projects, representing 20 industries and five continents. Respondents were asked about the challenges, tools and resources associated with the projects and the maturity of ML in their enterprise.
Results reinforced recent findings by MIT Sloan Management Review that large businesses with 100,000 employees or more are most likely to have an AI strategy, but only half do.
Other key findings include:
- 70% report that their first AI/ML investment was within last 24 months
- Over half of enterprises have undertaken fewer than four AI and ML projects
- Only half of enterprises have released AI/ML projects into production
- 78% of AI/ML projects stall at some stage before deployment
- 81% admit the process of training AI with data is more difficult than they expected
- 76% combat this challenge by attempting to label and annotate training data on their own
- 63% attempt to build their own labeling and annotation automation technology
- 71% of teams report that they ultimately outsource training data and other ML project activities