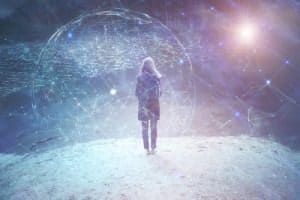
Why and how to get on board with the deep neural networks replacing traditional data models.
Deep neural networks are at the heart of a revolution changing the way every industry generates real-time predictions to run their businesses. Known by practitioners as “deep learning models”, they’re used in a wide range of artificial intelligence-like products such as self-driving cars and facial recognition scanners.
The term “AI” has been used so much when describing anything deep learning related that the two are nearly synonymous. (See here for more on avoiding the data science, machine learning, deep learning, AI taxonomy mess). Yet regardless of nomenclature, there’s no doubt: the “AI revolution” has some serious staying power.
See also: What We’re Learning About Deep Learning
A brief look at why this revolution is here to stay and a quick history of deep learning will set the context for three recommendations on how to take advantage of the AI revolution. In Part 2, a summary of the DL tool ecosystem will help you navigate this new world order.
Newcomer is here to stay
The “predictive analytics” revolution of the late 1990s morphed into the ”data science” revolution, which began around 2008. The data science wave is still going strong, has touched all industries. Its brought predictive analytics a real-time flavor, with applications such as online content and product recommendation, streaming sensor-based part-failure prediction, live-patient diagnosis, in-field resource optimization, and instantaneous fraud detection.
The “AI revolution” started about 2015. The original poster children of deep learning/AI included computer vision, language translation, and text mining. Today deep neural networks are replacing traditional data science models in use cases like recommendation engines, anomaly detection, and even time series analysis.
Why the takeover?
Deep learning models provide better accuracy, automate many of the mundane feature-engineering tasks of data scientists, and are constantly creating disruptive new applications. Future neural networks may write their own neural networks. In fact, if you ask an Amazon or Netflix engineer how many of their front-page machine learning recommendation algorithms have been replaced with deep learning models, the answer will be “All!”
A decade of hyper growth
Deep learning is advancing at a mind-bending pace and showing no signs of letting up. As recently as ten years ago, deep neural networks didn’t have the building blocks that make them so powerful today. ReLU, dropout, Adam optimization, and tools like TensorFlow all came into existence or popularity in the last five or ten years. Hard to believe, considering the large-scale adoption of deep learning today. A look at the evolution of neural networks provides context:
That’s a quick history of how we got to the present. The next question: How can organizations join the AI revolution today?
- Realize that your traditional real-time predictive scores can often be improved by leveraging deep learning models, especially if there’s a lot of data available to train new models. Invest in the education of your data scientists in deep learning methodologies. As a pilot project, encourage them to try to improve an existing model built on traditional machine learning. Hire a senior practitioner trained in deep learning to guide your team.
- Leaders need to stay up to date on specific deep learning use cases in their industry. They need to know how and where deep learning is being applied, both for incremental gains and for new disruptive applications. As you consider use cases, pay special attention to model explainability. Deep learning models are inherently black boxes. Model ethics, bias, and interpretability must be taken into account (see here and here for more).
- Set up your analytical teams for success by arming them with open source libraries running on an open source platform to future-proof against any single AI tool or cloud vendor. Time should be dedicated to staying up to date on the ecosystem. It is mostly an open source game. Things will continue to move too fast. You’ll want to be able to adopt promising technology as it emerges.
Next: Part 2- Summary of the deep learning tool ecosystem