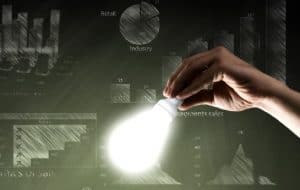
Consistently delivering high-quality data may seem daunting. But following these seven steps will help you manage the process.
High-quality data enables people to make great decisions at every level within an organization, from the sales and marketing departments to the C-suite. Yet, companies of all sizes struggle with data that’s out-of-date and unstructured.
Clearly, delivering high-quality data that can be trusted and used by all the appropriate stakeholders is no easy job. But it can be done. It’s a matter of methodically evaluating where the data is now, what needs to change, and why. Then you can develop plans and processes to systematically improve and maintain data quality.
This seven-step approach will make it possible to deliver high-quality data consistently.
1) Get the whole company involved. Data doesn’t just belong to the data department, or the sales team, or the marketing team. Data is a major asset that can—and should—be used by the entire organization. That’s why the first step toward consistently delivering high-quality data is getting the entire organization involved. The best way to do this is by creating a cross-functional data governance team made up of people from every relevant department. Depending on the organization, this might include people from sales, marketing, customer service, tech support, operations, and finance. A cross-functional data governance team will help you understand how each department—as well as the executive team—uses the data. To deliver high-quality data, you absolutely must understand what each of these stakeholders needs and why.
2) Determine which data is necessary. Now that you’ve got insight into data needs throughout the organization, it’s time to zoom in and apply that big-picture knowledge at the field level. While many people assume that more is better when it comes to data, that’s rarely the case. Instead, you need to make sure every field in each record serves an actual business use case. What’s more, you need to communicate that business use case to the people who actually touch the data. When people understand a data point’s purpose, they’re far more likely to enter that data correctly and completely.
3) Make an honest assessment of your database. Dig deep here. You need to figure out just how clean or dirty your data really is. Look for things like:
- Duplicates
- Records that can’t be contacted
- Typos
- Fake data, like “email@email.com”
- Sales opportunities showing up past their closed date
- Redundant fields that were created when two or more databases were merged.
4) Create a data backup plan. Before you start cleaning up your data, you’ll want to do a complete backup. In fact, it’s wise to perform on-demand backups before you make any major data changes, including merging new data into the system, in case you need to quickly reverse unwanted changes. You also will want to automate data backups on a regular schedule so you can recover quickly in the event of a data disaster. Therefore, you’ll need to determine how often you are going to back up data, how large a change merits an on-demand backup, and where you will securely store data backups.
5) Clean up your data. Now that you’ve uncovered the issues with your data and you’ve done a thorough backup, you’re ready to start tackling problem areas. Obviously, you can’t deliver high-quality data when your database is out-of-date and data decays rapidly. People change jobs. Companies move. Contact information changes, and on and on. Plus, data quality suffers when people—inside or outside your organization—make typos or enter dirty data. That’s why it’s vital to clean up, refresh, and verify your data.
6) Develop a data security model. This is critical. What steps are you currently taking to ensure the security of your data? Are they sufficient, or have circumstances changed? Does your industry require special data-privacy compliance measures? Also, consider data security from an inside-your-company perspective: According to the 2020 Verizon Data Breach Investigations Report, credential theft and phishing attacks to extract credentials from employees are among hackers’ most-employed tactics. As a result, it’s smart to limit the number of people in your organization who have access to the data. Determine who should be able to see records, edit records, and create new records. Make sure people cannot access—or affect—data they don’t need. This really is a win-win: Streamlining what users can touch and see makes the database easier to use, and it reduces data security risks.
7) Monitor and maintain data. Of course, it’s not enough to clean, refresh, and verify your data once. Keeping data clean, current, and complete is an ongoing activity. The key is to develop a strategic process for both monitoring and maintaining data quality. For example, whenever possible, you want to prevent bad data from entering the system. How might you accomplish that? What tools can you use to detect bad data—and repair it—on an ongoing basis? It is possible to automate a lot of data-quality tasks, including verifying data, deduplicating, managing imports, and standardizing data. This will make it easier to monitor and maintain data without dedicating your entire life to the process.
Consistently delivering high-quality data may seem daunting. But following these seven steps will help you manage the process. And, ideally, you’ll soon have other champions of data quality distributed throughout your organization, so you’re not driving quality initiatives on your own. After all, data is a major asset for your organization, but only when that data is clean and trustworthy.