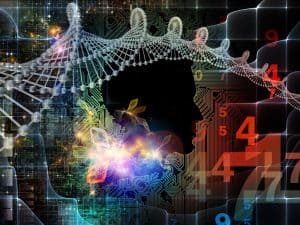
It is relatively common knowledge that AI systems can exhibit biases that stem from their programming and data sources.
We’ve all heard about the extraordinary complexity behind modern AI systems—GPT-3, for example, was built with 160-175 billion parameters—but how well do we understand what goes inside these systems? How well can we diagnose, and fix, what goes on in the “black box”?
That’s part of the problem that Anthropic, a public benefit corporation focused on AI safety and research, aims to solve. With the late April announcement of a $580M Series B fundraising round, led by Sam Bankman-Fried, CEO of FTX and including the Center for Emerging Risk Research (CERR), there’s a clear and present demand for these answers.
In a statement, Anthropic said they would use the funding to “build large-scale experimental infrastructure to explore and improve the safety properties of computationally intensive AI models.”
Anthropic co-founder and CEO Dario Amodei added: “With this fundraise, we’re going to explore the predictable scaling properties of machine learning systems while closely examining the unpredictable ways in which capabilities and safety issues can emerge at-scale. We’ve made strong initial progress on understanding and steering the behavior of AI systems and are gradually assembling the pieces needed to make usable, integrated AI systems that benefit society.”
See also: Algorithmic Destruction: The Ultimate Consequences of Improper Data Use
Are AIs inherently unsafe?
Potentially—but not in the way you might be thinking. Anthropic’s research doesn’t focus on the Hollywood-esque situations you might imagine, from HAL to Skynet, but rather more subtle and potentially pernicious ways that AI can be led astray by well-meaning researchers, data scientists, and users.
These mistakes can happen because of the sheer complexity of the systems. GPT-3 might have used hundreds of billions of parameters, but Google is training a new language model using more than a trillion, and GPT-4 could use hundreds of trillions of data points. No human or organization could ever hope to understand how these systems make their decisions or even define the boundaries of the decisions and outputs they might create.
That sets up a dangerous precedent as these models become more available for general use because the researchers who develop and maintain them are forever in a reactive mode. If they detect bias in the output, they can try more training or develop new guardrails, but the damage might already be done.
And according to Anthropic’s research, these problematic outputs from a large model can spring up after years of regular use. It only takes one edge-case question to open up Pandora’s box of unexpected results.
A recent report from NIST reflects that danger: “AI can make decisions that affect whether a person is admitted into a school, authorized for a bank loan or accepted as a rental applicant. It is relatively common knowledge that AI systems can exhibit biases that stem from their programming and data sources; for example, machine learning software could be trained on a dataset that underrepresents a particular gender or ethnic group.”
As a public benefit corporation—not one focused on profitability—Anthropic is focused on “making systems that are more steerable, robust, and interpretable.” In other words, the specific challenges that arise when you scale an extraordinarily complex AI.
For example, they’ve already figured out ways to mathematically reverse-engineer how small language models work, which could prove useful in the future for those who want to understand, with far more sophistication than we’re able to now, how something like GPT-3 arrives at its output. They’re also working on guardrails that help make larger language models, like GPT-4 or Google’s in-house development, more “harmless” to those who use them at a scale that could affect individuals and entire organizations or societies.
With this new influx of cash, Anthropic is expanding outward from its current team of 40 to develop new protocols for large-scale AI models with better safeguards. That reduces the reliance on reactive changes to problematic output, but perhaps even more importantly, they’re working on new tools that help researchers “peek” into AI systems to have confidence the safeguards are even working as expected.
Time will tell whether Anthropic can “catch up” to the pace of AI development from tech’s biggest companies and their wealth of computer power and trainable datasets. Still, a half a billion is undoubtedly a good start.