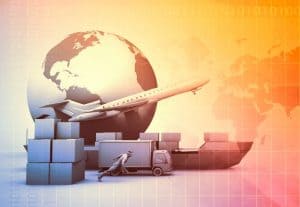
Analyzing aggregated data from different supply chain sources can give organizations a comprehensive view of their logistics networks.
Even before the challenges of 2020, the number of moving parts in supply chain management has made it a complicated business. Stakeholders at all levels have the tremendous responsibility of minimizing costs, optimizing inventory, and ensuring the quality, availability, and successful delivery of products. Moving forward, organizations are facing new issues, including inventory shortages and volatile customer demands due to the ongoing disruption caused by intermittent shutdowns.

Here’s where powerful, real-time data analytics can help.
For organizations looking for new ways to optimize their supply chain networks, incorporating advanced analytics of unstructured data and other innovations like the Internet of Things (IoT) and IoT edge computing into supply chain operations can help organizations gain in-depth insight into erratic supply and demand trends.
How analytics makes it better
A lack of end-to-end visibility across the supply chain leads to delays, unnecessary risks, disruptions, and increased costs. But gaining visibility isn’t always easy. Many organizations struggle to make sense of the huge amounts of data scattered across the supply chain network.
Supply chain analytics is the analysis of aggregated data from a variety of different sources, including application systems for procurement; inventory, order, and warehouse management; fulfillment; and transportation management (including shipping).
Part of the problem is that most of that data is unstructured, which makes it harder to organize, parse, and correlate than structured data. But within that data are the insights that can lead to today’s game-changing innovations.
Unstructured data can be spread across disparate systems, applications, and processes that don’t communicate with each other. It comes in different types, both files and objects. It could be a production image, handwritten text, an audio recording of a customer call, or raw sensor data from thousands of points across an organization’s ecosystem.

Today, technology is helping organizations across all industries extract the hidden value from their massive volumes of unstructured data. It just takes the right storage and management platform and advanced tools such as artificial intelligence and machine learning.
How to optimize your supply chain with analytics
The effort is worth it. Analyzing aggregated data from these different supply chain sources can give organizations a comprehensive view of their logistics networks. It can help them understand—and resolve—the effects of supply chain issues up and down the network.
Advanced methodologies like predictive analytics can help you accurately predict future trends, forecast inventory, and manage resources. Prescriptive analytics, on the other hand, answers the “what-if” questions that can help you make strategic supply chain planning decisions.
With more accurate data in hand, you can ask the strategic, analytical questions that will help you design the right strategies for optimizing your supply chain network. Here are five ways you can use analytics to optimize your supply chain.
1. Advanced demand forecasting
Efficient inventory management is critical to achieving supply chain optimization. Overstocks or stockouts of popular products can be a nightmare for suppliers. The inability to give a customer what they want when they want it can mean lost revenue as customers move on to other suppliers. Analytics used in inventory management for advanced demand forecasting can increase efficiency in several processes.
In a Logility survey, 36% of supply chain professionals indicated that optimizing inventory management to balance supply and demand was the main reason for adopting analytics. Real-time analysis of data on inventory, retail sales, and movement of goods provides actionable insights that can help organizations plan product promotions, determine price changes, and optimize inbound and outbound deliveries.
With real-time insights into inventory operations, you can optimize budgets and forecast how much inventory to keep on hand, improve inventory management with automated stock counts, and deliver inventory based on customer preferences and location to reduce distribution costs.

When you can create the optimal balance between supply and demand, you can ensure prompt delivery of services and products to customers with less lead time.
2. Predictive maintenance
By analyzing data from equipment and systems integrated with IoT, artificial intelligence, and machine learning, companies can conduct predictive maintenance to prevent downtime and improve safety in the manufacturing process. Predictive maintenance is a technique that analyzes historical and real-time data to detect possible failures in equipment and optimize schedules for preventive maintenance.
With a predictive maintenance strategy, maintenance is performed on equipment only when required. This helps you avoid unnecessary maintenance and the costs of spare parts and supplies that are associated with it. It also reduces downtime due to equipment undergoing maintenance and prevents unplanned maintenance that could cause delays in production and bottlenecks in the supply chain.
3. Increased warehouse efficiency
An efficient warehouse workflow process is crucial to ensuring that inventory is on hand to fulfill demand in the shortest time possible. Analytics at the warehousing stage of the supply chain can be used to help optimize and streamline warehouse operations.
More than two-thirds of organizations say they don’t have full visibility across their supply chains. Real-time analytical insights into the warehouse workflow give you visibility into the movement and condition of goods, show you how best to use your company’s warehouse space, and alert you to factors that could cause disruptions.
Goods sometimes need to be stored in a special way, based on temperature, fragility, or weight, for example. Unstructured data from IoT sensors and cameras used in the packaging process can ensure proper storage for perishable items.
Analyzing this unstructured data can help companies use their available storage capacity more efficiently, as well as better manage resources, including equipment, vehicles, and workers.
4. Real-time transportation tracking
Supply chain and logistics teams can use reporting analytics from their transportation management systems to make more informed decisions. For instance, data collected on traffic patterns on major roadways, weather conditions, and fuel consumption can help organizations optimize routes, minimize the frequency of expired and damaged goods, and reduce delivery times.
Companies can track cargo in real time at all stages of transportation, providing information that supports both decision-making and risk management. With this data, companies can keep their customers informed on changing delivery estimates in real time if delays occur.
Thanks to these large amounts of aggregated data, companies can identify and improve upon patterns of inefficiency—such as those that show repeated scheduling challenges or routes with constant traffic delays. Further, analytics can help companies determine the best inbound and outbound shipping routes by evaluating existing routes, backhaul routes, shipping scheduling techniques, and other constraints related to transportation.
5. Improve revenue, margins, and retailer satisfaction
Analytics can help with product placement, pricing, and promotions by providing insights about the supply and demand balance in different stores. Demographics, consumer behavior, and other trends and patterns also help organizations design their sales and marketing strategies to retain customers and increase revenue.
Data analysis at the point of sale also helps companies forecast demand based on locational, seasonal, and weather factors. With this data, retailers can understand the usage patterns and purchase habits of their customers, as well as how to prepare for peaks, set product inventory minimums, lower product recalls, and identify and cross-sell slow-moving stock.
