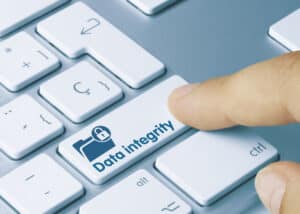
Knowing that modern data stacks will only grow more complex, companies must take steps to prevent loss of data integrity and ensure the efficacy of their data tools.
Due to its increasing complexity, the data stack is more divided than ever as data moves from source systems to data platforms to analytics platforms to prediction engines. And as a result of data’s continual ingestion and transformation in disparate tools, there are now far too many places and opportunities for data to lose its integrity—and, therefore, its value.
The growing complexity of the modern data stack
Regardless of where they are in terms of data maturity, most companies are trying to become more data-driven or more effectively use data. Because this is usually an in-depth process that occurs over time rather than in one singular initiative, we typically see data tools and platforms being incrementally brought into the data stack. In time, as needs change and technology evolves, tools and platforms may be swapped out or new ones added in.
Adding to this complexity is that few of these tools are able to handle more than one function. For instance, data from one source system usually must eventually be ingested into multiple disparate data platforms for use by different groups of business users. Or perhaps multiple source systems are used within one company, and that data must then be fed into numerous data platforms before it is then transferred to an analytics platform.
As data travels through this supply chain, because there are few options that cover multiple steps—let alone all of them—data must pass from one tool to another. Each of these transitions from one platform or tool to another introduces a new opportunity for data to be compromised. Knowing that modern data stacks will only grow more complex, how can companies take steps to prevent additional loss of data integrity and ensure the efficacy of their data tools?
In general, we see three key reasons for the loss of data integrity. While eliminating these causes is a challenge, there are fortunately some clever solutions to address these issues and ensure more successful data use and, ultimately, greater ROI.
Reason #1: The amount of transformation that data must go through
To become more data-driven and effective, companies need to be able to rely on data that are valid and accurate. Without that, they’ll see major impacts on business outcomes ranging from poor decisions to lost revenue. Consequently, it’s critical that the data they’re using has been verified to be correct at every stage in the data life cycle and data management journey—regardless of what tool or platform it’s in and how many transformations that data has gone through. This is especially critical for companies that rely on a greater number of disparate tools or platforms, which exponentially increases the number of transformations that data goes through. Companies and business users alike need greater assurance that the data they’re referring to down the line is the same as the data originally captured in source systems.
Solving this problem involves taking a proactive approach that focuses on identifying and resolving data accuracy and integrity issues at the earliest possible point. More specifically, I recommend integrating data integrity checks into the data life cycle itself starting from the very beginning. Build these integrity checks into every transition from one tool or platform to the next to introduce mechanisms that flag data issues or errors before they can be ingested into the next tool. By catching these issues early, companies can reduce the likelihood of poor data integrity—even as the number of data transformations steadily increases—before inaccuracies move downstream and cause greater negative business impact.
See also: Transformation Drivers: Innovative Data Management Tools and New Skillsets
Reason #2: Companies embarking on a data modernization journey without accounting for trust
Second, as companies become more data driven, they’re moving their data from legacy platforms to more modern platforms, hoping to get increased business value out of newer options. However, the value they see is only as good as the integrity of the data migration overall. After all, a more modern data platform’s success and adoption rates are directly proportionate to the trust that users have in the platform. Unless users know for sure that they can trust the data they have available in newer platforms, they’re unlikely to use it well if they use it at all, and companies will see reduced ROI from their recent platform additions.
So how do we provide users with the trust that they’re looking for? Across departments and user types, they’re looking for evidence that the data migration between a legacy platform or tool and a more modern option has happened effectively and correctly, with trustworthy processes in place to prove that their data is still correct. To address this challenge, I recommend introducing self-service auditing functionality to empower all users to perform data audits at scale.
Historically, there haven’t been self-service data integrity audits for users at large, as these tasks were mostly handled by the technical professionals handling the implementation. However, it’s become clearer than ever that there have to be solutions that empower business users to perform their own audit, as they’re often the ones with the experience and firsthand knowledge of the data necessary to know if it is indeed accurate. For instance, a developer performing a migration with validation processes of their own creation is unlikely to reassure a sales team that all of the customer data and lead information is complete and correct.
As companies are modernizing platforms, data integrity verification has a direct impact on the adoption of the platform and on the value the platform is adding, which makes self-service data integrity audits a must-have for successful platform use.
See also: What Are Data Contracts? And Should You Use Them?
Reason #3: Data innovations struggling to match the speed of changing business needs
And third, business needs are changing at an increasingly rapid pace—and that means data innovations need to be able to keep up. The speed at which we’re able to communicate, close deals, find new customers, roll out new products, and operate grows as people and business find new ways to work more efficiently. But as those business needs change, we often find that data tools and platforms are unable to keep up, plagued by slow implementation or ineffective testing processes.
Accelerating the pace of data innovation requires enabling changes to be made in lower landscapes and sent to production at a faster pace. And perhaps most critically, companies need automation of data testing. Automation of the ETL testing processes, regression testing, and data transformation testing have become front and center for achieving data innovations. This kind of methodology needs to be firmly established in the data organization and standardized as part of the innovation process to match the rate of business needs changing.
Ensuring data integrity for the long term
Data integrity is critical to business success, providing more accurate and complete data that enables better business decisions to be made regardless of what tool or platform they’re using. While compromised data integrity is a growing trend due to the increased complexity of the modern data stack, companies can build solutions to these issues into their systems by integrating data integrity checks throughout the entire data life cycle, introducing self-service auditing features for all users, and automating data testing to accelerate the pace of data innovations. In doing so, organizations can limit the loss of data integrity and achieve greater adoption rates and increased ROI.