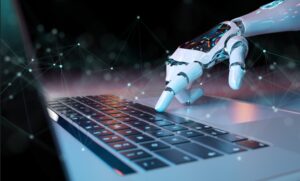
Leaders who embrace a hyperautomation strategy now will reap dividends, getting ahead of the competition in a world where customers of all types expect faster and smarter experiences more than ever.
Companies have been trying to automate their operations almost since the idea of a business was conceived. From Henry Ford’s car assembly lines more than 100 years ago to the introduction of retail barcodes later in the 20th Century, there’s been a steady evolution in manifesting business operations that do more, faster.
Today, we’re entering an era of hyperautomation, where everything possible is automated in a company. Artificial intelligence (AI) has the potential to evolve hyperautomation further than before—as long as organizations can figure out how to use it.
Business leaders understand the importance of accelerating automation. In a study last year, 96% of IT decision-makers and business leaders said automation is vital to digital transformation. One chief area of concern is process complexity, which describes a process that uses more complex logic than a simple sequence of steps and includes many different endpoints across various technologies or humans. According to the same study, process complexity hinders effective automation implementation, with 72% of IT decision-makers indicating increased difficulty managing real-world processes.
The good news is that AI should ease such difficulties and further overcome process complexity. With that in mind, here are three examples of how AI can power a hyperautomation strategy and cure process ills.
See also: The 2024 State of Automation: Are Leaders Ready to Ditch Legacy Thinking?
Modeling standard processes and generating business forms
With Predictive AI, businesses can analyze past process execution data to identify patterns and trends that can help optimize future processes, reduce costs, and enhance customer experiences. Business analysts and data scientists can use this data to train a new model or feed it into an existing model to predict future process instances using the existing execution data. For example, it’s possible to predict how long an instance will take to complete based on the tasks and variables in the process flow.
Generative AI has garnered a lot of headlines in the last two years because of its ability to generate content—most of it in the marketing realm. But the technology also holds great promise for business processes, particularly for modeling standard processes or the creation of business forms.
Generative AI can model standard processes to create accurate representations of business workflows. This involves understanding and mapping out the steps involved in these processes. By doing so, businesses can streamline operations, improve efficiency, and ensure consistency in the execution of these processes. Additionally, generative AI can aid in the creation of business forms, further enhancing operational efficiency.
Business forms may not be as intriguing to the general public as text-to-video apps, but they should grab the attention of IT leaders. AI form generation is a game-changer, enabling users to create forms up to 10 times faster by simply inputting natural language prompts. This approach ensures that forms are generated within the business context, accurately describing the use case. The outcome is a meticulously crafted layout with form widgets for checklists, drop-down menus, and date and time components.
Of course, generative AI automations are all about speed and efficiency. For example, an auto rental company could create a generative automation prompt such as “I need a car damage claim form.” The forms would be automatically created as one step in the automated process and delivered to the correct contact.
Optimizing process decisions with A/B testing
I recently spoke with an IT leader who mentioned the intriguing idea of A/B testing AI-powered decisions against DMN decisions. This can enable CIOs, enterprise architects, and developers to drive some decisions using DMN, specifically with terministic business rules, and compare these to AI-powered decisions.
A/B testing can help them nimbly choose which brings more speed to their automation, optimizing processes one at a time. It underscores that technologists and others should always pressure-test their assumptions.
In practice, by leveraging A/B testing to refine AI models and compare them against traditional methods like DMN, an insurance company can, for example, significantly enhance its risk assessment capabilities and operational efficiency, ultimately leading to a stronger competitive position in the market. So, I believe we’ll see more A/B testing of AI decisions versus DMN outcomes.
All in all, AI offers great promise to company executives looking to make processes more efficient by accelerating automation. It’s early days, but companies are already using AI for automation, and if they haven’t started, now is the time. Leaders who embrace a hyperautomation strategy now will reap dividends, getting ahead of the competition in a world where customers of all types expect faster and smarter experiences more than ever.