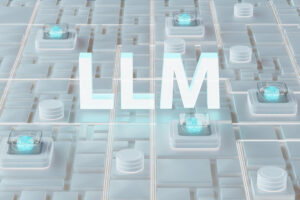
The accelerated adoption of large language models (LLMs) is shifting from experimentation to operationalization, fueled by cost reductions, advancements in symbolic knowledge generation, and a focus on energy-efficient AI.
As we look ahead this year, a transformative wave is set to reinvent how enterprises leverage Generative AI (GenAI). The accelerated adoption of large language models (LLMs) is shifting from experimentation to operationalization, fueled by cost reductions, advancements in symbolic knowledge generation, and a focus on energy-efficient AI. As a result, businesses will unlock new opportunities to utilize AI, from processing vast volumes of unstructured text with agents to fine-tuning smaller models for specific tasks.
From on-prem to cloud-native AI, towards zero-cost token generation
In the early days of Generative AI (GenAI), there were significant concerns about privacy and data leaks. Companies pushed towards on-premises hosting of language models. Given the shortage of GPU supply that needed to catch up significantly with the demand, the cost of hosting and operating LLMs made intelligent application development difficult and expensive. On the other hand, LLM-as-a-service companies not only improved the inference times and throughput but also got into a race to the bottom for the price of token generation. At the same time, data cloud providers like Snowflake have invested in building their GenAI stack and providing security and privacy guarantees. In 2025, the cost reduction will push the development of simple LLM workloads, such as entity linking into production.
Enterprise agents devour vast volumes of text
Riding on the wave of cheap token generation, enterprises have terabytes of text waiting to be mined to drive better decisions. In the first big data wave that rose in early 2010, companies started mining volumes of generated untouched stored data once the hardware became cheap and ML tools were developed. The conditions for GenAI seem ripe now, so communication data, such as email, Zoom transcripts, Slack messages, Jira tickets, etc., will be consumed massively by agents in the new year that can provide analytics insights and decision support. Imagine a CRO in an organization with hundreds of complex sales trying to get the accounts’ status and progress. The daily standup meetings were different leads reporting details of each project will be replaced by agents providing dashboards, charts, and alerts with actionable items.
More symbolic knowledge generation
Knowledge Graphs (KG) are the backbone of modern enterprise efficiency. However, for many years, building one was expensive. Language models have been proven to be an excellent assistant for building KGs. Human supervision is still required. The biggest problem has been the motivation for starting the process. Companies can only afford to make a knowledge graph by tying it to an application. Usually, a significant upfront effort is required to build a high-quality, clean version of a KG to start driving an application. GraphRAG is a popular application that can work with an inexact version of a KG and simultaneously deliver value. GraphRAG quickly provides a KG that companies can use to iterate and perfect over time. As mentioned in the previous section, in 2025, agents will process massive volumes of textual information and convert unstructured text to symbolic facts as part of the knowledge graph.
See also: Navigating the AI Landscape: Why Multiple LLMs Are Your Best Bet
The dawn of fine-tuning
While approaching the limits of in-context learning, academia, and industry are exploring the value of fine-tuning more. While question answering is well handled with in-context methods like RAG and its variants, there are cases where latency and speed matter, so fine-tuning smaller models makes more sense. Also, we saw LLMs able to solve complicated reasoning problems that can be toys for the moment, like playing chess, solving sudokus, and other puzzles. There are a lot of enterprise applications, such as planning supply chain optimization, that are based on the same principles. While we expect to see a small adoption in the new year, more exploration and interest will shift toward this paradigm. While there seem to be many lower-hanging fruits, we shouldn’t exclude the possibility of an explosion of use and adoption of LLM applications like this, given the availability of a currently idle tech workforce.
The year of Binary (no multiplication) LLM?
It is no secret that LLMs consume vast amounts of energy. While there is a lot of interest in expanding to more energy production, such as more efficient nuclear energy, research pursues improvements in hardware and algorithms. The most expensive operation is floating-point multiplications. While some recent research has demonstrated the implementation of neural networks with addition only, we are still waiting to see it in production. On the other hand, we have seen more progress towards the 1-bit transformer, which requires only bitwise operations that are superfast and energy efficient. While possible for several years, they always needed to improve performance. The gap seems to narrow. Will 2025 be the year of the binary transformer? If yes, expect to see it on devices you have never imagined!
The long tail implications of energy-efficient LLMs
AI companies heavily invest in clean energy, focusing mainly on the nuclear option. The same level of infrastructure overinvestment was seen in the nineties from telecom companies in fiber networks, which led to the telecom crash in 2000. While devastating, the thousands of miles of fiber networks sold at a low cost eventually to the web 2.0 companies and fueled the growth we saw in the past 20 years. Imagine a technology that reduces the energy cost of token generation by two orders of magnitude. This will cause a surplus of green energy from the Tech companies that can accelerate the green energy transition for the governments.